PROJECT PROPOSALSENTIMENTAL ANALYSIS OF TWITTER DATASET USING PYTHONCONTENTS 1. Introduction2. Problem Statement3. Statement of Need4. Literature Review5. Methodology6. Programming Languages7. Expected Results8. Timeline9. ReferencesINTRODUCTION Microblogging website has become the most important source to find out all kind of information that the user needs. This is due to the real-time nature of microblogs where people post a variety of opinions on diverse topics. The topics vary from discussing current political issues to finding the rating of products based on the opinions. These opinions can be used to make different conclusions on different sectors of the modern world. But, finding the solutions based on this data is not an easy task. We have to depend on different sentimental analysis methods to find the solution. [1]. (Columbiauniversity-paper, 2019)The project on Sentimental Analysis of Twitter data; based on each of the tweets on a taxi service running in a country, the best features can be identified.PROBLEM STATEMENT The famous microblog Twitter is used to build a model that classify the data or merely called tweets into different sentiments based on their polarity. The sentiments are categorized as positive, negative or neutral. The analysis of data is achieved by a two-level mechanism. In the first level, word analysis occurs where each word is taken to determine the polarity. In the second level, the sentence is taken and analysis occurs by combinations of different words.In our project, we use this sentimental analysis for identifying the taxi service Uber. Different features like payment, service, cancellation and safety are taken to find the best feature. Python is used in our project in the prediction of real-time Twitter data based on pattern classification and data mining algorithms.STATEMENT OF NEED Data-driven techniques are the most powerful as well as necessary methods in taking decisions on the real world problems. We use the sentimental analysis in almost all fields like politics, health care, customer ratings and so on. So, there is a need to build algorithms that apply data mining techniques in the collection of opinion based data.The project on analysis of Uber data from Twitter will help the company to find the good and bad features of the service. This will help the company to make improvements in giving the best customer service.LITERATURE REVIEW Twitter is one of the most popular site where people respond to a variety of matters with strong viewpoints. Hence people have used the sentimental approach in Twitter data in various projects.[2]Data Mining Approach For Classifying Twitters Users, a project done by Mashael Saeed Alqhtani and M. Rizwan Jameel used the sentimental approach. They classify social groups as terrorist and dissident so that it is possible to identify the anomalous user as soon as possible. For this first need to extract a certain set of features that are used to characterize each group using different data mining technique and these data are stored in the database. For this extraction sentiment analysis, text mining and opinion mining are used. The main goal is to calculate the similarities of selected user tweets with respect to features that are extracted. For this analysis, they are using Python Twitter Tools (PTT) called Tweepy. It is possible to detect anomalous groups by analyzing text from Twitter ( Data Mining Approach For Classifying Twitters Users, 2019).[3] Muqtar Unnisa, Ayesha Ameen and Syed Raziuddin proposed Opinion Mining on Twitter Data using Unsupervised Learning Technique where the Twitter data set is clustered into positive and negative clusters using unsupervised machine learning technique like spectral clustering. The result of the analysis is represented as a scatter plot graph and hierarchical graph. They are using a spectral algorithm that reduces the eigen values for the similarity matrix and plotting the graph by using the K means algorithm. They also mention hierarchical clustering as another way to plot the graph by using the data points. From their analysis, they conclude that unsupervised learning can be used to solve the domain dependency. (Opinion Mining on Twitter Data using Unsupervised Learning Technique, 2019)METHODOLOGY STEP 1: Dataset CollectionTwitter dataset or the tweets are collected using Twitter API.Data collected based on particular keywords on Uber.Example: payment, price, cancellation, safety.Done using Python library tweep.STEP2: Data CleansingTweets are tokenized. (NLTK Library)Removal of symbols like @ or # and URLs.Removal of Stop words.Lexical Normalization is done.Done using Python library numpy and nltk.STEP3: Sentimental AnalysisClassify based on three sentimental labels: positive, negative, neutralNaïve Bias Algorithm used to predict the sentiments.Done using Python library textblob. STEP 4: VisualizationData visualization done by using matplotlib library of python or WordCloud. ? DATA SET: Data set from Twitter containing Uber tweets.? ALGORITHM: Naïve Bias Algorithm ? TOOLS: Python, Pycharm ? PROGRAMMING LANGUAGE: PythonEXPECTED RESULTS The sentimental analysis of Twitter dataset on Uber taxi service is taken to find the polarities. Based on these polarities we can determine the feature that best describes its service. TIMELINE REFERENCES [1] Sentiment Analysis of Twitter Data. Apoorv Agarwal ,Boyi Xie, Ilia Vovsha, Owen Rambow, Rebecca Passonneau. Department of Computer Science ;Columbia University, New York, NY 10027 USA.[2] Data Mining Approach For Classifying Twitters Users. International Journal of Computer Engineering & Technology (IJCET) Volume 8, Issue 5, Sep-Oct 2017, pp. 4253, Article ID: IJCET_08_05_006. By Mashael Saeed Alqhtani and M. Rizwan Jameel Qureshi, Faculty of Computing and Information Technology, King Abdul-Aziz University, Jeddah, Saudi Arabia.[3] Opinion Mining on Twitter Data using Unsupervised Learning Technique. International Journal of Computer Applications (0975 8887) Volume 148 No.12, August 2016. By Muqtar Unnisa, Ayesha Ameen and Syed Raziuddin IT Dept Deccan College of Engineering and Technology Darussalam Hyderabad.
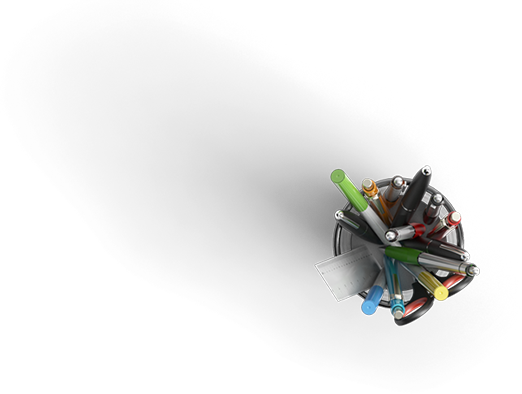



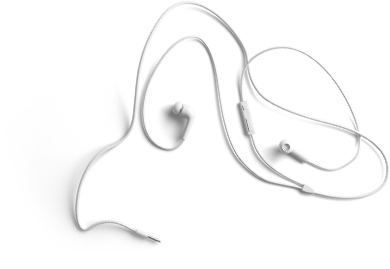
Sentimental Analysis of Twitter Dataset Using Python
Mar 17, 2020 | Information Technology
Our Service Charter
1. Professional & Expert Writers: Topnotch Essay only hires the best. Our writers are specially selected and recruited, after which they undergo further training to perfect their skills for specialization purposes. Moreover, our writers are holders of masters and Ph.D. degrees. They have impressive academic records, besides being native English speakers.
2. Top Quality Papers: Our customers are always guaranteed of papers that exceed their expectations. All our writers have +5 years of experience. This implies that all papers are written by individuals who are experts in their fields. In addition, the quality team reviews all the papers before sending them to the customers.
3. Plagiarism-Free Papers: All papers provided by Topnotch Essay are written from scratch. Appropriate referencing and citation of key information are followed. Plagiarism checkers are used by the Quality assurance team and our editors just to double-check that there are no instances of plagiarism.
4. Timely Delivery: Time wasted is equivalent to a failed dedication and commitment. Topnotch Essay is known for timely delivery of any pending customer orders. Customers are well informed of the progress of their papers to ensure they keep track of what the writer is providing before the final draft is sent for grading.
5. Affordable Prices: Our prices are fairly structured to fit in all groups. Any customer willing to place their assignments with us can do so at very affordable prices. In addition, our customers enjoy regular discounts and bonuses.
6. 24/7 Customer Support: At Topnotch Essay, we have put in place a team of experts who answer to all customer inquiries promptly. The best part is the ever-availability of the team. Customers can make inquiries anytime.
Recent Posts
- Positive Psy-5.1 Discerning Truth Getting Started
- Given your understanding of the functions of the different lobes of the brain, how might a person be affected by damage to the temporal lobe?
- History Discussion Assignment
- Discussion: Explore how lifestyle changes-such as nutrition–can prevent or modify disease processes
- Read the article on Spirit Airlines
Archives
- January 2025
- August 2024
- July 2024
- June 2024
- May 2024
- April 2024
- January 2024
- December 2023
- August 2023
- July 2023
- June 2023
- May 2023
- January 2023
- October 2022
- September 2022
- August 2022
- July 2022
- June 2022
- May 2022
- March 2022
- February 2022
- January 2022
- December 2021
- November 2021
- October 2021
- September 2021
- August 2021
- July 2021
- June 2021
- May 2021
- April 2021
- March 2021
- February 2021
- January 2021
- December 2020
- November 2020
- October 2020
- September 2020
- August 2020
- July 2020
- June 2020
- May 2020
- April 2020
- March 2020
- February 2020
- January 2020
- December 2019
- November 2019
- October 2019
- September 2019
Categories
- `
- .uul l illl'
- "" ""Qualitative Analysis Assignment: Transcript 3
- "" which is attached to this assignment.
- (15 points) Lot Ai? ?Xn be a random sample from a f/(0,0) population with unknown parameter 0 > 0. Find the likelihood ratio test (LRT) of size a for testing Ho : 0 > 0q .vs. H : 0 < Oq.
- { ""cell_type"": ""markdown""
- /files/-np-ex19-7b-xlsx
- /files/0886109913504154-pdf,/files/1363460706065057-pdf,/files/0886109914560741-pdf
- /files/11357-txt,/files/cavell-pdf
- /files/20191118150623part-vii-assessment-tool-development-rub1-pdf
- /files/20191119051422iom-report-future-of-nursing-rubric-xlsx
- /files/20200428035024defend-or-refute-guide-pdf
- /files/adjustmentdisorder-pdf,/files/depression1-pdf-6973733
- /files/assignment1j-docx-7815535,/files/assignment2j-docx
- /files/assignment2-docx-5634861,/files/networking14-pkt,/files/20200204-routers-table-docx,/files/20200204-network-infrastructure-design-pdf-5640375
- /files/assignment2-docx-8830717
- /files/bookaccess-docx-5088805
- /files/bookchap1-412-pdf,/files/bookchap1-412-pdf-7977187
- /files/businessculturaldimensionsanalysisgradingrubric-pdf-7789673"
- /files/casestudy2-docx-5603093
- /files/chapter5-pdf-7822037
- /files/crjs3001wk1studentreplies-docx
- /files/developingobjectives1-docx,/files/article3assignments3-41-pdf,/files/developingobjectives1-docx-7919359
- /files/discussionreflectionguide-docx,/files/introduction-to-criminology-10th-edition-9781544339023-pdf,/files/sample-docx-7598097
- /files/essay2-documentedargumentrevised22-pdf-6947741
- /files/frenchandindianwar-docx-7926377,/files/bostonteapartyaftermath-docx
- /files/homework2negotiationmorgan-docx,/files/homeworknegotiation1michele-docx
- /files/labassignmnetw8-docx-7665133
- /files/lessonweek4-docx
- /files/mat240houselistingpricebyregionpjct5-xlsx-7794861,/files/mat240modulefiveassignmenttemplatepjct5-docx-7794863
- /files/microm53question-docx
- /files/milecture-pdf,/files/motivationalinterviewing-thebmj-pdf
- /files/nrnpprac6635comprehensivepsychiatricevaluationtemplate-docx-8263023"
- /files/principlesofcorporatefinance13erichardbrealey-pdf-7629311,/files/wk71-docx,/files/wk72-docx,/files/wk73-docx,/files/wk74-docx
- /files/problemstatementchecklist-pdf-6967979,/files/week4article-pdf-6967981
- /files/prospectusfinaldraft-correctedversion-docx,/files/dissertationprospectusoriginalversion-docx
- /files/reflectionpaperguidelines-docx-7570139
- /files/rubricpandv-pdf-7584061,/files/page11-pdf-7584063,/files/page9-10-pdf-7584065,/files/implicationofpiagetsandvygotskytheories-summer2021socialstudiesmethodsforece-fieldece-3571-01-pdf
- /files/screenshot2021-02-27at11-35-38am-png"
- /files/sec-516-t6-meetingtheneedsofdiverselearners-docx"
- /files/spd-400-d-t6-studentgoalsandinterventionplantemplate-docx-7938583,/files/rub-docx-7938623
- /files/taskdifficultyandincubation1-docx
- /files/unitvinternationlstudyguide-pdf-7796117
- /files/visualcommunicationassessment23051-docx
- /files/week3assignmentg-docx
- /files/week4rubric-docx-7856191
- /files/week7readingdestructivebehavior-pdf-7890761,/files/bibliu-print-9781452236315table143-pdf
- /files/westernphilosophyananthologybycottinghamjohnz-lib-org-pdf"
- /files/woodch9-pdf,/files/chapter8-pdf-6111751
- /files/youthexposedtoviolenceindc-august2021-docx,/files/chapteronethenatureandtoolsofresearch-pdf
- ~I'vcrn I""theorems"" follow from it
- 1111 writing and reading is embedded in some Discourse
- 12 font
- 12 point font
- 2015
- 2021
- 2021). HTML pages are an excellent example that allows network shares hence makes the embedding of the resources possible. Therefore
- 3 PAGE ESSAY ON WHY I DONT WANT ANY TATTOOS. I NEED CLEAR EXAMPLES
- 4-6sentence · Sentence1-2:Author + Genre + Context of the Bible book (that the story is a part of) · Sentences 3-5: Brief Summary Including Story Genre (historical narrative, mythology, or parable).
- 4)."
- Acc 206
- ACC 206, BUSINESS FINANCE
- ACC201
- ACC544, BUSINESS FINANCE
- ACC556
- ACC573, Business & Finance
- ACC60171, Other
- Accounting & Finance
- Acct 101
- ACCTG406
- ACIS5104
- ACOL202
- acquire (at least) one initial Discourse. This initial Discourse
- actively engaging with course materials
- acts
- AD712, Business & Finance
- adding beliefs
- ADMIN565
- ADMN575, OTHER
- allocating specific time slots for coursework
- ALY6100
- American Military University
- AMERICAN MILITARY UNIVERSITY, BUSINESS FINANCE
- AMERICAN MILITARY UNIVERSITY, WRITING
- American Public University System
- AMERICAN PUBLIC UNIVERSITY SYSTEM, BUSINESS FINANCE
- AMERICAN PUBLIC UNIVERSITY SYSTEM, HUMANITIES
- AMH2020
- analyze and evaluate the following questions:
- and cost were controlled.
- and academic grammar and usage.
- and architecture the guys work execution at the program or system level. At the risk of falling victim to stating the obvious
- and describe the type of economic analysis that you would use in the evaluation.
- and external resources recommended by instructors. Utilize online libraries
- and interactive components. Take comprehensive notes
- and managing your time effectively
- and often write
- and personal health record (PHR)
- and related terms for two separate concepts. (examples: technology-computer
- and Transportation and Telecommunication. Using The World Factbook
- and users are able to inject SQL commands using the available input (Imperva
- ANM104
- ANM104 OL1
- ANTH130, SCIENCE
- ANTHROP 2200
- Anthropology 130
- Applied Science
- Applied Sciences
- Applied SciencesApplied Sciences
- Architecture and Design
- Architecture and DesignArchitecture and Design
- Arizona State University
- ARIZONA STATE UNIVERSITY, WRITING
- Art
- ART101
- ART2010
- ARTH102
- article
- Arts
- ARTS1301
- ASC400, BUSINESS FINANCE
- ashford university
- ASHFORD UNIVERSITY, BUSINESS FINANCE
- ASHFORD UNIVERSITY, HUMANITIES
- ASHFORD UNIVERSITY, OTHER
- ASHFORD UNIVERSITY, SCIENCE
- Ashworth College
- asking thoughtful questions and providing constructive feedback to your peers. Regularly check your course emails and notifications
- assignments
- at least
- Atlantic International University Online, Science
- attitudes
- BADM735
- BAM515, BUSINESS FINANCE
- BCJ3601, BUSINESS FINANCE
- BCN4431
- BEHS380, WRITING
- Berkshire Community College
- Bethel University
- BETHEL UNIVERSITY, HUMANITIES
- beyond the family and immediate kin and peer group. These may be 1111.
- BHR3352
- BHR3352 Human Resource Management
- BIO1100
- BIO1408
- BIO2401
- BIO3320
- BIO354, SCIENCE
- BIOCHEM202
- Biology
- Biology – Anatomy
- Biology – AnatomyBiology – Anatomy
- Biology – Ecology
- Biology – Physiology
- BIOLOGY 10, SCIENCE
- BiologyBiology
- Blog
- BME351
- body
- Bowie State University
- Bowie State University, Science
- brings with it the (poten- u.il) acquisition of social ""goods"" (money
- BROCK UNIVERSITY, BUSINESS FINANCE
- BROCK UNIVERSITY, OTHER
- BROOKDALE COMMUNITY COLLEGE, HUMANITIES
- BROOKLYN COLLEGE, WRITING
- BUAD326, Business & Finance
- BULE303
- BUS1001
- BUS120, Business & Finance
- BUS125, WRITING
- BUS187, Business & Finance
- BUS232
- BUS242
- BUS303, BUSINESS FINANCE
- BUS410, BUSINESS FINANCE
- BUS472, SCIENCE
- BUS475
- BUS499
- BUS520, Business & Finance
- BUS530
- BUS542
- BUS599
- BUS620
- BUS623
- BUS630
- BUSI320
- Business
- Business – Management
- Business & Finance
- Business & Finance – Financial markets
- Business & Finance – Financial marketsBusiness & Finance – Financial markets
- Business & Finance – Marketing
- Business & Finance – MarketingBusiness & Finance – Marketing
- Business & Finance – Supply Chain Management
- Business & Finance , BUS430
- Business & Finance , BUSN370
- Business & Finance , COMM240
- Business & Finance , COMS2302
- Business & Finance , ENT527
- Business & Finance , FIRE3301
- Business & Finance , G141COM1002
- Business & Finance , GB520
- Business & Finance , GB540
- Business & Finance , IBSU487
- Business & Finance , JWI515 Managerial Economics
- Business & Finance , MGT16
- Business & Finance , MGT496
- Business & Finance , MGT498
- Business & Finance , MGT521
- Business & Finance , MT460
- Business & Finance , PM586
- Business & Finance , RMI3348
- Business & Finance , SOC450
- Business & Finance , south university online
- Business & Finance , Strayer University
- Business & Finance , University of Phoenix
- Business & Finance , Wilmington University
- Business & Finance, Trident University
- Business & FinanceBusiness & Finance
- Business and Finance
- Business Finance – Accounting
- Business Finance – AccountingBusiness Finance – Accounting
- Business Finance – Economics
- Business Finance – EconomicsBusiness Finance – Economics
- Business Finance – Management
- Business Finance – ManagementBusiness Finance – Management
- Business Finance – Operations Management
- Business Finance – Operations ManagementBusiness Finance – Operations Management
- BUSINESS FINANCE, CBBU1001
- BUSINESS FINANCE, COLORADO STATE UNIVERSITY GLOBAL
- BUSINESS FINANCE, COLORADO TECHNICAL UNIVERSITY
- BUSINESS FINANCE, COLUMBIA SOUTHERN UNIVERSITY
- BUSINESS FINANCE, COM 510
- BUSINESS FINANCE, CRJ101
- BUSINESS FINANCE, DOC660
- BUSINESS FINANCE, EASTERN KENTUCKY UNIVERSITY
- BUSINESS FINANCE, ECN 501
- BUSINESS FINANCE, ECO2251
- BUSINESS FINANCE, ECO531
- BUSINESS FINANCE, FIN 500
- BUSINESS FINANCE, FIN31FMS12019
- BUSINESS FINANCE, GRANTHAM UNIVERSITY
- BUSINESS FINANCE, HLS3302
- BUSINESS FINANCE, HRC164
- BUSINESS FINANCE, HRM 500
- BUSINESS FINANCE, INDS 400
- BUSINESS FINANCE, INT113
- BUSINESS FINANCE, INTL3306
- BUSINESS FINANCE, ISDS 351
- BUSINESS FINANCE, LAWS OF EVIDENCE
- BUSINESS FINANCE, LIBERTY UNIVERSITY
- BUSINESS FINANCE, MAN3504
- BUSINESS FINANCE, MBA 5121
- BUSINESS FINANCE, MG260
- BUSINESS FINANCE, MGMT386
- BUSINESS FINANCE, MGT 521
- BUSINESS FINANCE, MGT211
- BUSINESS FINANCE, MICHIGAN STATE UNIVERSITY
- BUSINESS FINANCE, MIDDLE TENNESSEE STATE UNIVERSITY
- BUSINESS FINANCE, MKT331
- BUSINESS FINANCE, MKT419
- BUSINESS FINANCE, NORTHEAST MONTESSORI INSTITUTE
- BUSINESS FINANCE, OAKLAND UNIVERSITY
- BUSINESS FINANCE, PARK UNIVERSITY
- BUSINESS FINANCE, RASMUSSEN COLLEGE
- BUSINESS FINANCE, SOUTHERN NEW HAMPSHIRE UNIVERSITY
- BUSINESS FINANCE, TRIDENT INTERNATIONAL UNIVERSITY
- Business Strategy
- C11E
- Calculus
- California Baptist University
- California Coast University
- CALIFORNIA STATE UNIVERSITY NORTHRIDGE, OTHER
- can be studied
- can never 21 really be liberating literacies. For a literacy to be liberating it must contain both the Discourse it is going to critique and a set of meta-elements (language
- Capella University
- Capella University, Humanities
- Capital L. George Adams
- CATEGORY
- CE304
- CE445
- CEE792
- CEGR338
- Chamberlain College of Nursing
- Chapter 3
- Charles R Drew University of Medicine and Science
- charles sturt university
- Chaudhary Charan Singh University, Humanities
- CHEM101
- CHEM111
- CHEM1411, Science
- CHEM202, Science
- CHEM210, Science
- CHEM410
- Chemistry
- Chemistry – Chemical Engineering
- Chemistry – Organic chemistry
- Chemistry – Pharmacology
- Chemistry – Physical chemistry
- ChemistryChemistry
- Childcare
- CHMY373, SCIENCE
- Choose three problematic issues that are currently facing older people living in the community?
- CINE286U
- CIS210
- cis273
- CIS359
- CIS510, Other
- CIS524
- CIVL6603, Science
- CJ430, SCIENCE
- CJA444
- CJUS300, Other
- Classics
- CMIT495
- CMSC140
- Colorado Christian University
- COLORADO STATE UNIVERSITY GLOBAL, SCIENCE
- COLORADO STATE UNIVERSITY, SCIENCE
- Colorado State UniversityGlobal
- Colorado Technical University
- COLORADO TECHNICAL UNIVERSITY, OTHER
- Colorado Technical University, Programming
- Columbia Southern University
- COLUMBIA SOUTHERN UNIVERSITY, OTHER
- Columbia Southern University, Science
- COLUMBIA SOUTHERN UNIVERSITY, WRITING
- Commerce
- Communication
- Communications
- COMMUNITY-BASED CORRECTIONS
- COMP1007
- Computer Science
- Computer Science – Java
- Computer Science- Python
- Computer ScienceComputer Science
- Construction
- correctness
- Cosc1437
- counseling chemical dependency adolescents
- Criminology
- CRJ305
- cross-site scripting
- CS101
- CSIT 100, PROGRAMMING
- CSPM326
- CST 610, PROGRAMMING
- Cultural Studies
- culturally appropriate intervention to address childhood obesity in a low-income African American community.
- CUR535
- CUYAMACA COLLEGE, HUMANITIES
- CUYMACA COLLAGE, HUMANITIES
- Data Analysis
- DAVIDSON COLLEGE, OTHER
- defensive programming allows for more efficient processes while also protecting systems from attack.
- DES201
- Describe the difference between glycogenesis and glycogenin ? Explain in 10 to 12 lines.
- Design
- Digital Marketing Plan for Nissan Motor Co. The plan will identify the current marketing opportunity and/or problem(s) and propose digital marketing solutions. Please use header in the attachment."
- Discuss one way in which the Soviet Union fulfilled communist thought, and another way in which it did not with reference to O'Neil's Chapter 9.
- Discuss the pros and cons of free-market based economies and how they impact the modern, globalized economy? What comes to your mind when you hear the term "globalization?"
- Dissertation
- DMM612, Science
- DMM649, SCIENCE
- Draft and essay of 1,000 words minimum, stating the Most Important and Relevant aspects to be considered when carrying on INTERNATIONAL NEGOTIATIONS or MULTI-CULTURAL NEGOTIATIONS.
- Drama
- each time a user extracts the ZIP file
- Earth Science – Geography
- Earth Science – GeographyEarth Science – Geography
- Earth Science – Geology
- EAS1601
- ECD 541, HUMANITIES
- ECE 452
- Ecommerce
- ECON335
- Economics
- ECPI University
- EDUC696
- Education
- EducationEducation
- EEL3472C
- EEL3705
- EET110
- EFFAT UNIVERSITY JEDDAH, HUMANITIES
- elasticity
- ELI2055A
- EMDG 230, SCIENCE
- Emglish
- Emory University
- Employment
- EN106
- EN106, HUMANITIES
- EN109
- EN206, HUMANITIES
- ENC1102, Writing
- eng 100
- ENG100
- ENG101
- ENG101, Humanities
- ENG102
- ENG102, Humanities
- eng106
- ENG1102, WRITING
- ENG124
- ENG124, Humanities
- ENG124, Writing
- ENG1340, HUMANITIES
- ENG200, Humanities
- ENG207
- eng2206
- ENG2211
- ENG305
- ENG812
- Engineering
- Engineering – Chemical Engineering
- Engineering – Civil Engineering
- Engineering – Civil EngineeringEngineering – Civil Engineering
- Engineering – Electrical Engineering
- Engineering – Electrical EngineeringEngineering – Electrical Engineering
- Engineering – Electronic Engineering
- Engineering – Mechanical Engineering
- Engineering – Mechanical EngineeringEngineering – Mechanical Engineering
- Engineering – Telecommunications Engineering
- EngineeringEngineering
- ENGL 120
- ENGL 124, OTHER
- ENGL 124, WRITING
- ENGL 2030, HUMANITIES
- ENGL1102
- ENGL120, HUMANITIES
- ENGL120SP2019, WRITING
- ENGL126
- ENGL1302
- ENGL130E, HUMANITIES
- ENGL147N, HUMANITIES
- ENGL2, Humanities
- English
- English – Article writing
- English – Article writingEnglish – Article writing
- English Language
- English Literature
- EnglishEnglish
- ENGR350
- ENST202CORE274
- ensuring you allocate dedicated time for coursework
- Environment
- Environmental Science
- Environmental Sciences
- Environmental Studies
- especially for a small company
- Essay Writing
- etc¦). Please note at least five organizational activities and be specific when responding.
- ETH321
- ETHC445N
- Ethnic Studies 101
- European Studies
- EXNS6223
- Family
- Fashion
- February 20). What is defensive programming? EasyTechJunkie. Retrieved December 30
- film industry
- FILM INDUSTRY, HUMANITIES
- Film Studies
- FIN 500
- FIN330, MATHEMATICS
- FIN370
- Final Essay
- Find the uniform most powerful level of alpha test and determine sample size with the central limit theorem
- Florida International University
- Florida National University
- Florida State College at Jacksonville
- FoothillDe Anza Community College District
- Foreign Languages
- Foreign Languages – Spanish
- formulations
- from https://www.pcmag.com/news/fat32-vs-ntfs-choose-your-own-format
- G124/enc1101
- Gallaudet University
- General Studies
- General_Business
- GEO1206
- GEOG100, Science
- Geography
- GEOL3200, HUMANITIES
- Geometry
- George Mason University
- GERM1027
- GERO 101, SCIENCE
- GERON101
- GLG101, Science
- GO16
- Government
- GovernmentGovernment
- GOVT2305
- GOVT2305, Humanities
- GOVT2306
- Grand Canyon University, Science
- Grand Canyon University, Writing
- Grantham University
- GRANTHAM UNIVERSITY, PROGRAMMING
- GRANTHAM UNIVERSITY, WRITING
- GROSSMONT COLLEGE, HUMANITIES
- Grossmont-Cuyamaca Community College District
- GROSSMONT-CUYAMACA COMMUNITY COLLEGE DISTRICT, HUMANITIES
- GU299, WRITING
- Hawaii Pacific University
- HC310
- HCA415
- HCA521
- HCM550, SCIENCE
- Hcs370
- HCS446
- he focused on aspects of the U.S. that combined democratic and increasingly capitalist characteristics. THINK ABOUT the points De Tocqueville made.
- Health & Medical
- Healthcare
- HIM 2588, MATHEMATICS
- HIM 500, SCIENCE
- HIM301
- HIS 108
- HIS101
- HIS105
- HIS200
- HIST104A, Humanities
- HIST111
- HIST1301, HUMANITIES
- HIST1302
- HIST1320
- HIST1700
- HIST2620
- HIST350, Humanities
- HIST405N, HUMANITIES
- HIST459, Humanities
- History
- History – American history
- History – American historyHistory – American history
- History – Ancient history
- History – Ancient historyHistory – Ancient history
- History – World history
- History – World historyHistory – World history
- HISTORY4250, Humanities
- HistoryHistory
- HLSS508, OTHER
- HMP403
- Hospitality
- HOST1066, WRITING
- Housing
- How do the changes in ship technology effect port operations? Discuss at least 3 factors contributing to port operations and development. Address cargo and passenger liners.250 words
- How have Mary Calderone, SIECUS and other sex educators changed how sex education is perceived? (100 words minimum)
- HOWARD UNIVERSITY, SCIENCE
- HR Management
- HRM300
- HRT6050, Writing
- HSA305
- HSA535
- HSC3201
- HSN476
- HUM1002
- HUM115
- HUM115, Writing
- Human Resource
- Human Resource Management
- Human Resource ManagementHuman Resource Management
- Human Resources
- HUMAN RESOURCES DEVELOPMENT AND MANAGEMENT, SCIENCE
- Human Rights
- HUMANITIES
- Humanities, Alcorn State University
- HUMANITIES, HY 1110
- Humanities, LMC3225D
- HUMANITIES, LONG BEACH CITY COLLEGE
- HUMANITIES, MUSIC1306
- HUMANITIES, OAKLAND COMMUNITY COLLEGE
- HUMANITIES, PH 100
- HUMANITIES, POINT LOMA NAZARENE UNIVERSITY
- HUMANITIES, PRINCE GEORGE'S COMMUNITY COLLEGE
- Humanities, PSY105
- HUMANITIES, PSY330 THEORIES OF PERSONALITY
- Humanities, PSYC 1101
- HUMANITIES, PSYCH305
- HUMANITIES, PSYCH635 PSYCHOLOGY OF LEARNING
- HUMANITIES, RSCH8110
- HUMANITIES, SAN DIEGO STATE UNIVERSITY
- HUMANITIES, SAN JACINTO COLLEGE
- Humanities, SOC1010
- HUMANITIES, SOC401
- HUMANITIES, SOCIOLOGY OF RELIGION
- HUMANITIES, SOUTHERN NEW HAMPSHIRE UNIVERSITY
- HUMANITIES, STRAYER UNIVERSITY
- HUMANITIES, SWK110
- HUMANITIES, UNIVERSITY OF CALIFORNIA
- HUMANITIES, UNIVERSITY OF CALIFORNIA IRVINE
- HUMANITIES, UNIVERSITY OF HOUSTON-DOWNTOWN
- Humanities, University of Maryland University College
- i need the attached work to look like this. please redo and make it look like this.
- I need these questions answered fully. I have the assignment and the notes attached for it. Do not use chegg or course hero. This is due Wednesday 4/14 at 10:00 pm which is almost 4 full days. Thanks!
- I need to re organize a research paper I attached all my information and I attached you an example how is going to be. Please follow the instruction and the references has to be APA 7edition
- Identify a cardiac or respiratory issue and outline the key steps necessary to include for prevention and health promotion
- identify the leadership theory that best aligns with your personal leadership style
- if you suggest trying to do this
- IGLOBAL UNIVERSITY
- IHS2215
- Iii Mlch
- III nuistery of such superficialities was meant to
- Implement classifiers KMeans, Random Forest and Decision Tree, SVM,XGBoost and Naive Bayes for the given dataset of audio samples to findout top genre for an audio sample(which one fits best)
- In a cardiac issue what are the key steps necessary to include for prevention and health promotion.
- in any other way
- include a paragraph about which side of the case a forensic psychologists might support and why.
- indeed
- India
- INDIANA UNIVERSITY BLOOMINGTON, SCIENCE
- INF690
- INF690, Other
- Information Systems
- Information SystemsInformation Systems
- Information Technology
- INSTITUTE OF PUBLIC ADMINISTRATION SAUDI ARABIA, PROGRAMMING
- INT700, OTHER
- International Business
- International Relations
- International Studies
- Internet
- Introduction to Biology
- Is jury nullification sometimes justifiable? When?
- ISSC351
- It Research
- IT380
- IT550, Business & Finance , Southern New Hampshire University
- ITC3001
- ITP120
- ITS 631, PROGRAMMING
- ITS835, Other
- JEDDAH COLLEGE OF ADVERTISING, WRITING
- Journalism
- KNOWLEDGE IS POWER, OTHER
- Languages
- Law
- Law – Civil
- Law – CivilLaw – Civil
- Law – Criminal
- Law – CriminalLaw – Criminal
- LawLaw
- Leadership
- lecture slides
- Leisure Management
- Liberty University
- LIBERTY UNIVERSITY, WRITING
- lIlgll.Igt· (1II1In·d
- Linguistics
- literacy is always plural: literacies (there are many of them
- Literature
- Literature Review
- Literature review funnel on "cyber security"
- LiteratureLiterature
- MA105
- MAJAN COLLEGE, WRITING
- Management
- Manpower
- Marketing
- Math
- MATH 1030
- MATH144, MATHEMATICS
- Mathematics
- Mathematics – Algebra
- Mathematics – Calculus
- Mathematics – Geometry
- Mathematics – Numerical analysis
- Mathematics – Precalculus
- Mathematics – Probability
- Mathematics – Statistics
- Mathematics – StatisticsMathematics – Statistics
- Mathematics – Trigonometry
- MATHEMATICS, MGT3332
- Mathematics, National American University
- Mathematics, PSY325
- MATHEMATICS, PUBH8545
- Mathematics, QNT275
- MATHEMATICS, STAT 201
- MBA503
- McMaster University
- ME350B, SCIENCE
- MECH4430, SCIENCE
- Mechanics
- Media
- Medical
- Medical Essays
- MGMT2702
- MGMT410
- MGT173, SCIENCE
- MHR6451
- MIAMI UNIVERSITY, WRITING
- Military
- Military Science
- MKT501
- MKT690, OTHER
- MN576
- MN581
- MN610, SCIENCE
- MNGT3711
- Music
- MVC109
- N4685
- NATIONAL INSTITUTES OF HEALTH, SCIENCE
- NATIONAL UNIVERSITY, SCIENCE
- Needs to be at least 300 wordswithin the past five years.No plagiarism! What key aspects do you believe should guide ethical behavior related to health information, technology, and social media?
- no workable ""affirmative action"" for Discourses: you can't 19 Ill' let into the game after missing the apprenticeship and be expected to have a fnir shot at playing it. Social groups will not
- Northcentral University
- not writing)
- nothing can stand in her way once she has her mind set. I will say that she can sometimes be hard headed
- Nova Southeastern University
- NR447, SCIENCE
- NRS429VN
- NRS44V, OTHER
- NRS451VN
- NRSE4540
- NSG426
- NSG486
- NSG6102
- NSG6102, SCIENCE
- Numerical Analysis
- NUR231NUR2349, SCIENCE
- NUR647E
- NURS350
- NURS508
- NURS6640
- Nursing
- NURSING LEADERSHIP AND MANAGEMENT, SCIENCE
- NursingNursing
- Nutrition
- offering learners the flexibility to acquire new skills and knowledge from the comfort of their homes. However
- OHIO UNIVERSITY, SCIENCE
- Online Discussion Forums Grade and Reflection Assignment : Current Topic Artificial Intelligence HR Planning Career and Management Development Labour RelationsForum
- operation security
- Operations Management
- or do those companies have an ethical obligation to protect people? In this assignment
- ORG5800, OTHER
- Organisations
- OTHER
- Other, PAD631
- OTHER, PARK UNIVERSITY
- OTHER, PLA1223
- Other, POLI330N
- OTHER, PROFESSIONAL NURSING NU231 NUR2349
- Other, RTM404
- OTHER, SAINT LEO UNIVERSITY
- OTHER, SOC3210C1
- Other, SOCW6333
- OTHER, SOUTHERN NEW HAMPSHIRE UNIVERSITY
- Other, The University Of Southern Mississippi
- OTHER, TRIDENT UNIVERSITY INTERNATIONAL
- Other, UC
- OTHER, UNIVERSITY OF MARYLAND UNIVERSITY COLLEGE
- OTHER, UNIVERSITY OF SOUTH FLORIDA
- Other, Walden University
- paying attention to grammar and spelling. Actively participate in discussions
- Personal Development
- PhD Dissertation Research
- PHI 413V, SCIENCE
- Philosophy
- Photography
- PHY290
- PHYS204L206
- Physics
- Physics – Astronomy
- Physics – Electromagnetism
- Physics – Geophysics
- Physics – Mechanics
- Physics – Optics
- PhysicsPhysics
- Physiology
- PNGE332, SCIENCE
- Political Science
- Political SciencePolitical Science
- Politics
- PowerPoint slides
- privacy
- PROFESSIONAL NURSING NU231 NUR2349, SCIENCE
- PROFESSIONAL NURSING NU231NUR2349, SCIENCE
- Programming
- Programming , College of Applied Sciences
- PROGRAMMING, STRAYER UNIVERSITY
- PROGRAMMING, WILMINGTON UNIVERSITY
- Project Management
- proper grammar
- Protein
- provide a discussion on what could have been done better to minimize the risk of failure. If you have not yet been involved with a business process redesign
- PSYC8754, WRITING
- Psychology
- PsychologyPsychology
- PUB373, SCIENCE
- Purdue University
- Rasmussen College
- Read a poam and write a paragraph to prove "The table turned".
- Reading
- ReadingReading
- readings
- Reference this
- REL1030
- Religion
- RES861, Science RES861
- Research Methodology
- Research methods
- Research Proposal
- Research questions
- Retail
- Rutgers university
- SAFE4150
- safety statutes
- Santa Clara University
- SCI 220, SCIENCE
- SCI115, SCIENCE
- Science
- Science, Strayer University
- SCIENCE, THOMAS JEFFERSON UNIVERSITY
- SCIENCE, WEST COAST UNIVERSITY
- SCIENCE, WEST TEXAS A & M UNIVERSITY
- Sciences
- SCM371, Writing
- Search in scholarly sources the similarities and difference between PhD and DNP. Post three similarities and three differences found on your research. Do not forget to include your reference.
- Security
- self-actualization
- several things can happen
- Should the government operate public transportation systems?250 words
- so that it is not biased?
- so too
- SOC 450
- Social Policy
- Social Science
- Social Science – Philosophy
- Social Science – PhilosophySocial Science – Philosophy
- Social Science – Sociology
- Social Science – SociologySocial Science – Sociology
- Social Sciences
- Social ScienceSocial Science
- Social Work
- Society
- Sociology
- someone cannot engage in a Discourse in a less than fully fluent manner. You are either in it or you're not. Discourses are connected with displays of
- SP19, WRITING
- SPC2608
- SPD310
- Sports
- Statistics
- succeeding in online courses requires a different approach compared to traditional classroom settings. To help you make the most of your online learning experience
- such as notifications from social media or email. Organize your study materials and have a reliable internet connection to ensure seamless access to course materials.
- Technology
- that personal ethics and organizations ethics are two different and unrelated concepts. Others
- the attribute is useful
- The directions are attached. However you must read the PDF file first in order to answer the questions.
- the role of work and money
- Theatre
- then reply to a minimum of 2 of your classmates' original posts.
- Theology
- Threat of artificial intelligence 800 words.
- to be true of second language acquisition or socially situ ated cognition (Beebe
- to better promote the value and dignity of individuals or groups and to serve others in ways that promote human flourishing.
- to usc a Discourse. The most you can do is III It'! them practice being a linguist with you.
- total fat consumption
- Tourism
- Translation
- Transportation
- U110
- Uncategorized
- University of Central Missouri
- University of South Florida
- UNIVERSITY OF SOUTH FLORIDA, WRITING
- Video Games
- Watch this meditation https://www.youtube.com/watch?v=doQGx4hdF3M&feature=youtu.be and write a one page reflection
- WCWP10B
- we can always ask about how much ten- 12 """""" or conflict is present between any two of a person's Discourses (Rosaldo
- What approaches to the study of poverty does economic sociology offer? More specifically, what might sociologists studying poverty focus on besides poor households, neighborhoods, and individuals?
- What is the philosophical matrices for Behaviorism, Constructivism, and Reconstructivism
- What key aspects do you believe should guide ethical behavior related to health information, technology, and social media?
- what place did government have to improve markets? What does the concept of ""crowding out"" mean in all of this?
- What should be done to maintain optimum stock levels and why is it important to keep accurate and up-to-date records of stock in medical practice?
- whether good or bad. The intent of the short research projects is to dig a little deeper into some of the topics
- which triggers the vulnerability. As soon as the user downloads this shortcut file on Windows 10; windows explorer will
- Would somebody read these quotes and answer those three questions at the bottom for me?Disregard number two I will look for myself in the text.
- Write short essay based on evidence about the 2 cons of Sex Education 250-300 words 2 reference minimum no plagiarism
- WRITING
- writing assignment, you will analyze asymmetric and symmetric encryption. Evaluate the differences between the two of them and which one that you would determine is the most secure.
- Writing, Personal Code of Technology Ethics
- you believe you can provide the CIO with the information he needs.
- you will learn how to search for scholarly
- you will need to read the TCP standard. TCP was first defined in RFC 793. A link to this document is provided. https://tools.ietf.org/html/rfc793
- Young People
Recent Comments