???Evaluation of Machine Learning Algorithms for Classifying Deep Brain Stimulation Respective of On and Off StatusAbstract Essential tremor is a prevalent neurodegenerative movement disorder. Deep brain stimulation represents a highly effective means of treatment, especially for scenarios for which medical intervention is no longer feasible. One of the major post-operative challenges is the determination of an optimal set of tuning parameters. Optimizing the deep brain stimulation parameters can impart a time-intensive task to the clinician. The smartphone in the context of a wearable and wireless inertial sensor system offers the capability to objectively quantify the characteristics of the tremor. Machine learning in conjunction with a wearable and wireless inertial sensor system, such as a smartphone, can distinguish between disparate states, such as deep brain stimulation in On and Off status. Multiple machine learning classification techniques are available, such as the multilayer perceptron neural network, support vector machine, K-nearest neighbors, logistic regression, J-48 decision tree, and random forest. The objective of this research is to evaluate these six machine learning classification algorithms for classification of deep brain stimulation regarding On and Off status for Essential tremor during a reach and grasp task. The reach and grasp task is quantified through the smartphone as wearable and wireless inertial sensor system mounted to the dorsum of the hand and secured by latex glove. Multiple feature set scenarios are considered, such as recordings from both the accelerometer and gyroscope, accelerometer, and gyroscope. These scenarios facilitate the determination of the most robust machine learning algorithms. The multilayer perceptron neural network, support vector machine, K-nearest neighbors, and logistic regression achieve the highest classification accuracy for three feature set scenarios: derived by recordings from both accelerometer and gyroscope, accelerometer, and gyroscope.I. INTRODUCTIONEssential tremor is a predominant neurodegenerative movement disorder that is relatively more prevalent than Parkinsons disease. Contrasting to Parkinsons disease, Essential tremor also is represented through both resting tremor and kinetic tremor, which involves tremor during intentional movement [1, 2]. The tremor characteristics of these movement disorders has been successfully quantified through the application of wearable and wireless inertial systems [3, 4, 5, 6, 7, 8, 9, 10, 11, 12, 13]. In particular these wearable and wireless systems have been instrumental for the objective quantification of response to therapeutic intervention through the application of deep brain stimulation, such as On and Off status, through the distinction of machine learning classification [11, 12, 13].Deep brain stimulation offers a highly efficacious therapy intervention for the amelioration of movement disorder tremor symptoms [14]. The deep brain stimulation system is surgically implanted through stereotactic surgery by a skilled neurosurgeon [14, 15]. Another highly challenging task is the acquisition of the optimal parameter configuration [16, 17].The clinician is tasked with a substantial number of parameter configurations, which consist of a myriad of settings regarding electrode polarity, amplitude, pulse width, and simulation frequency. Intuitively the convergence to an optimal configuration can present a time intensive and laborious endeavor [15, 16, 17]. Another matter of concern is in light of whether the temporal snapshot of a clinical meeting properly addresses the inherent variations observed during a neurodegenerative movement disorder.Two technologies offer considerable potential for advancing the efficacy of deep brain stimulation tuning optimization: wearable and wireless systems and machine learning. Wearable and wireless systems through their inertial sensor systems can quantify movement disorder tremor, which can be consolidated into a feature set for machine learning classification [11, 12, 13]. However, a challenge with the proper application of machine learning is the determination of the most appropriate machine learning algorithm [18, 19, 20]. There are many available machine learning algorithms for the scope of this research endeavor, and the following are under consideration: multilayer perceptron neural network, support vector machine, K-nearest neighbors, logistic regression, J-48 decision tree, and random forest. The objective of the research endeavor is to evaluate these six machine learning algorithms for the classification of deep brain stimulation using On and Off status for Essential tremor during a reach and grasp task that is measured by a smartphone as a wearable and wireless inertial sensor platform to establish a quantified feature set.II. BackgroundA. Fundamental characteristics of Essential tremorEssential tremor and Parkinsons disease are contrasted as disparate regarding the nature of their respective movement disorder [1, 21, 22]. Parkinsons disease involves resting tremor, which can be measured with the patient sitting in a chair [8, 13]. However, Essential tremor is comprised of both resting and kinetic tremor, and kinetic tremor is observed while the subject conducts a voluntary movement task [1, 2]. For example, a reach and grasp task of a lightweight object on a table can satisfactorily demonstrate kinetic tremor, which can be recorded by the inertial sensor signal of a wearable and wireless system, such as a smartphone [11, 23].For approximately the last two millennia there have been attempts among the medical community to ameliorate the kinetic tremor morbidities evident for this type of movement disorder. Further effort has emphasized the evolution of diagnostic acuity for Essential tremor [1, 24]. However, the neuroanatomical origins of Essential tremor have not been conclusively determined from a medical perspective. Contemporary positions attribute the origins of Essential tremor to the central nervous system, such as degeneration about the cerebellar pathways [1, 25].The tremor frequency regarding Essential tremor has been established as inversely proportional relative to age. The symptoms are more prevalently revealed by kinetic tremor compared to resting tremor. Kinetic tremor generally displays a frequency on the order of 4 Hz to 12 Hz [1].B. Conventional therapy for the treatment of Essential tremorMedical therapy through drug intervention is the traditional strategy for mitigating the influence of Essential tremor symptoms. Two widely applied drugs are propranolol and primidone. However, with the neurological origins of the movement disorder not conclusively determined, the efficacy of a specific medical treatment can display variable degrees of success for a number of patients [1, 26].For some scenarios pharmacotherapy is determined to be intractable for treating Essential tremor, and irreversible neurosurgery becomes an inevitable contingency [1]. The thalamotomy is an irreversible neurosurgical procedure that disrupts pathways associated with the thalamus. The Gamma Knife applies radiosurgery that can achieve this alternate [27, 28].C. Deep brain stimulation for treatment of Essential tremorDeep brain stimulation offers considerable advantage for the treatment of Essential tremor. Intuitively this treatment does not have the permanency of ablative techniques. Deep brain stimulation is effectively a reversible procedure. If the device is considered not effective for the individualized treatment of Essential tremor, then the deep brain stimulation system can be removed. Furthermore, the deep brain stimulation system is equipped with a considerable array of parameter configurations, which enables highly individualized therapeutic intervention [29].The target selection for the anatomical structure of the deep brain to be treated is dependent to the unique movement disorder scenario that is being treated. Regarding Essential tremor the ventral intermediate nucleus (VIM) is the prevalent target. The VIM receives pathways from the cerebellum and projects to the motor cortex [30, 31].The deep brain stimulation system is an integral device that consists of multiple components and subsystems. The component that interacts directly with the deep brain structure is the electrode lead. The electrodes are prolonged through connecting wire that merges with the implantable pulse generator. An internalized battery provides the energy source for the implantable pulse generator. The implantable pulse generator consists of the electronic circuitry that provides the stimulating electric signal, which stimulates the targeted aspect of the deep brain to treat Essential tremor [15]. The skilled implantation of a deep brain stimulation system by an expert neurosurgeon only represents a preliminary aspect of properly treating a person with Essential tremor through this novel form of therapy [17].There are multiple parameters that are adjusted to establish a configuration, and current deep brain stimulation systems applied four parameters:amplitudefrequencypulse widthelectrode polarity [15, 16]Acquiring the optimal tuning configuration, which is imperative for efficacious intervention, presents a time-consuming and challenging task. Given the considerable array of parameter configurations, a systematic approach would be beneficial in light of the limited medical resources available [17]. A quantified rather than subjective form of feedback to ascertain the efficacy of a deep brain stimulation system tuning configuration may considerably advance the optimization process.D. Wearable and wireless systems for quantifying movement disorderThe application of wearable and wireless systems to advance diagnostic acuity by the feedback acquired through the signal of an inertial sensor has been advocated as a means to objectively quantify movement disorder status [3, 4, 5, 6, 7]. During 2010 LeMoyne et al. applied a smartphone as a functional wireless accelerometer platform to quantify Parkinsons disease tremor, which is another type of movement disorder [8]. One of the immediately discernible benefits for the application of wearable and wireless systems, such as the smartphone, is the observation that experimental and post-processing resources can be remotely situated anywhere in the world [4, 5, 6, 7].The smartphone is equipped with an inertial sensor package that consists of both an accelerometer and gyroscope. A software application enables the smartphone to function as a wireless inertial sensor platform. The signal data is recorded and attached to an email for wireless transmission using the Internet. In essence, this email resource is representative of a functional Cloud computing resource [4-7, 32].Subsequent evolutions of the role of wearable and wireless systems, such as the smartphone, pertained to the evaluation of deep brain stimulation efficacy for Essential tremor. The smartphone with its inertial sensor package quantified Essential tremor attributes for a reach and grasp task during the scenarios of deep brain stimulation On and Off modes. The signal data was consolidated into a feature set for machine learning classification, and considerable classification accuracy was attained differentiating between On and Off modes [11, 12]. A similar technique was recently applied for Parkinsons disease hand tremor [13]. However, the appropriateness of a machine learning algorithm for classification is contextually unique to the experimental research conditions [33].Fig. 1. Mounting of the smartphone about the dorsum of the hand through a latex glove for reach and grasp of a lightweight object.Fig. 2. Deep brain stimulation systems patient programmer.The current research objective is to evaluate the classification accuracy for an assortment of machine learning algorithms to distinguish between deep brain stimulation using On and Off modes for a subject with Essential tremor. Six machine learning algorithms have been selected for this research endeavor:Multilayer Perceptron Neural NetworkSupport Vector MachineK-Nearest NeighborsLogistic RegressionJ-48 Decision TreeRandom ForestThe feature set for machine learning classification is established by consolidating the inertial signal (accelerometer and gyroscope) obtained through a smartphone functioning as a wearable and wireless inertial sensor platform.III. Materials and MethodsThe application of multiple machine learning algorithms to establish classification accuracy for distinguishing between deep brain stimulation On and Off status was conducted from the perspective of engineering proof of concept. One subject with Essential tremor being treated with deep brain stimulation was selected. Informed consent was established through the Institutional Review Board provided by Allegheny General Hospital of Pittsburgh, Pennsylvania. Tremor characteristics were acquired through the use of a smartphone.The smartphone utilized a software application that enabled the simultaneous recording of both the accelerometer signal and gyroscope signal. The inertial sensors for the smartphone recorded signal data at a rate of 100Hz for approximately 10 seconds. The data was attached to an email for wireless transmission using the Internet for remote post-processing. The smartphone was mounted to the dorsum of the hand and secured by a latex glove. Figure 1 shows a representative picture of the strategy for mounting the smartphone. Figure 2 displays the deep brain stimulation systems patient programmer.The six machine learning algorithms were provided by Waikato Environment for Knowledge Analysis (WEKA) using ten-fold cross validation. Software automation through Matlab consolidated the inertial signal data acquired by the smartphone into a feature set using an Attribute-Relation File Format (ARFF). The feature set was composed of five attributes maximum, minimum, mean, standard deviation, and coefficient of variation of the roll, pitch, and yaw components of the gyroscope signal and the acceleration magnitude. These feature set attributes have been successfully applied for multiple machine learning classification scenarios pertaining to wearable and wireless inertial sensors [12, 13, 34, 35, 36, 37].The following experimental protocol was applied to acquire 10 trials while the deep brain stimulation system was set to On mode and 10 trials in Off mode for a subject with Essential tremor.Experimental Protocol:1. Mount the smartphone to the dorsum of the hand using a latex glove.2. Initiate the smartphone for the accelerometer and gyroscope recording countdown.3. Instruct the subject to reach and grasp a lightweight object (rolled flexible bandage) on a table after the audio tone indicates the signal recording has commenced.4. Transmit the recorded inertial signal data for post-processing using wireless Internet connectivity.IV. Results and DiscussionThe deep brain stimulation system notably mitigated tremor for the Essential tremor subject using the On mode by contrast to the Off mode. The suppression of tremor is discernible with the deep brain stimulation system set to On compared to Off. The inertial sensors of the smartphone provide objectively quantified definition of the nature of the tremor. Figure 3 demonstrates the acceleration magnitude for the subject while conducting the experiment using On mode. Tremor is notably amplified for figure 4, which represents the acceleration magnitude for the Essential tremor subject with deep brain stimulation set to Off status.Fig. 3. Acceleration magnitude of Essential tremor subject conducting a reach and grasp task with deep brain stimulator set to On status.Fig 4. Acceleration magnitude of Essential tremor subject conducting a reach and grasp task with deep brain stimulator set to Off status.Four inertial sensor signals were applied to develop the feature set: acceleration magnitude and roll, pitch, and yaw components of the gyroscope signal. Regarding the four inertial sensor signals five attributes were applied to each respective signal:MaximumMinimumMeanStandard deviationCoefficient of variationThe experimental data consisting of 10 trials with the deep brain stimulation system set to On and 10 trials with the deep brain stimulation system set to Off. Software automation using Matlab consolidated the experimental data to a feature set for machine learning classification. The Waikato Environment for Knowledge Analysis known as WEKA enabled machine learning classification through the following six machine learning algorithms: multilayer perceptron neural network, support vector machine, K-nearest neighbors, logistic regression, and J-48 decision tree, and random forest.The machine learning classification accuracy for differentiating between deep brain stimulation set to On and Off modes using the smartphone as a wearable and wireless inertial sensor to provide quantified feedback for all six machine learning algorithms is summarized in Figure 5. In particular, multilayer perceptron neural network, support vector machine, K-nearest neighbors, logistic regression, and J-48 decision tree achieved 100% classification accuracy. Random forest misclassified one deep brain stimulation Off mode instance as On mode attaining 95% classification accuracy.The future objective of the use of a wearable and wireless inertial sensor with deep brain stimulation is to enable automated closed loop parameter optimization using the objective and quantified feedback from a wearable and wireless inertial sensor system. The Food and Drug Administration of the United States considers the deep brain stimulation system to be a Class 3 device [38]. The Class 3 device is considered as greatest risk to the patient [39]. Intuitively the machine learning classification response according to multiple inertial sensor quantification scenarios would be warranted. In order to further determine the most appropriate machine learning classification algorithm, further investigation of the classification accuracy of respective of only the accelerometer signal and only the gyroscope signal is warranted. Essentially, this extension of the machine learning classification endeavor seeks to determine the most robust machine learning classification algorithm in the event that one of the sensors has a failure mode issue.Figure 6 provides the classification accuracy of distinguishing between deep brain stimulation On and Off modes for the Essential tremor subject with the feature set constrained to only the acceleration magnitude attributes. The J-48 decision tree achieved 100% classification accuracy. All of the five other machine learning classification algorithms (multilayer perceptron neural network, support vector machine, K-nearest neighbors, logistic regression, and random forest) attained 95% classification accuracy, as a consequence of misclassifying one deep brain stimulation Off mode instance as On mode.Using only the attributes derived from the gyroscope signal data further machine learning classification accuracy for differentiating between deep brain stimulation On and Off modes for the Essential tremor subject was determined as illustrated in figure 7. Interestingly the J-48 decision tree only attained 90% classification accuracy using only the gyroscope signal data. One instance was misclassified as deep brain stimulation Off mode instead of On mode, and another instance was misclassified as On mode instead of Off mode. The five other machine learning classification algorithms (multilayer perceptron neural network, support vector machine, K-nearest neighbors, logistic regression, and random forest) attained 100% classification accuracy.Given the machine learning classification accuracy findings presented in figures 5, 6, and 7 the multilayer perceptron neural network, support vector machine, K- nearest neighbors, and logistic regression achieve the highest classification accuracy for three feature set scenarios: both accelerometer and gyroscope, accelerometer, and gyroscope. The future goal is to integrate one of these machine learning classifiers in to an embedded system using the objective quantified feedback from a wearable and wireless inertial sensor system for the close-loop and automated parameter optimization of deep brain stimulation for the treatment of movement disorder, such as Essential tremor. Future studies are also recommended for the more refined selection of an appropriate machine learning algorithm using a feature set derived from quantified feedback from a wearable and wireless inertial sensor system, such as computational efficiency in consideration of the processing capability of the embedded system of close-loop and automated parameter optimization of deep brain stimulation.V. ConclusionFig 5. Machine learning classification accuracy for differentiating between deep brain stimulation set to On and Off modes using both smartphone accelerometer and gyroscope.Fig 6. Machine learning classification accuracy for differentiating between deep brain stimulation set to On and Off modes using smartphone accelerometer.Fig 7. Machine learning classification accuracy for differentiating between deep brain stimulation set to On and Off modes using smartphone gyroscope.Essential tremor is a prevalent form of neurodegenerative movement disorder. The research objective is to assess the capabilities of six machine learning algorithms for the classification of deep brain stimulation using On and Off status for a subject with Essential tremor conducting a reach and grasp task. The future goal is to integrate the machine learning algorithm into a closed-loop system for automated parameter optimization of the deep brain stimulation for the broad treatment of movement disorders. Six machine learning algorithms were evaluated: multilayer perceptron neural network, support vector machine, K-nearest neighbors, logistic regression, J-48 decision tree, and random forest. The nature of the movement disorder was objectively quantified through a smartphone representing a wearable and wireless inertial sensor equipped with both an accelerometer and gyroscope. The inertial sensor signal data was consolidated into a feature set for machine learning classification. The research addressed three feature set scenarios, such as both the accelerometer and gyroscope, accelerometer, and gyroscope. Addressing these feature set scenarios enables a more informative determination of the most robust machine learning algorithms available. Four machine learning algorithms attained the highest classification accuracy regarding the three feature set scenarios: multilayer perceptron neural network, support vector machine, K-nearest neighbors, logistic regression.In pending studies, it is recommended that the machine learning algorithms are also contrasted for computational efficiency in consideration of the available embedded system processing capacity. The future goal is to integrate machine learning with an embedded system while using quantified feedback from the wearable and wireless system to objectively assess the response to the closed-loop automation of optimizing a parameter configuration for deep brain stimulation treatment of movement disorder, such as Essential tremor.
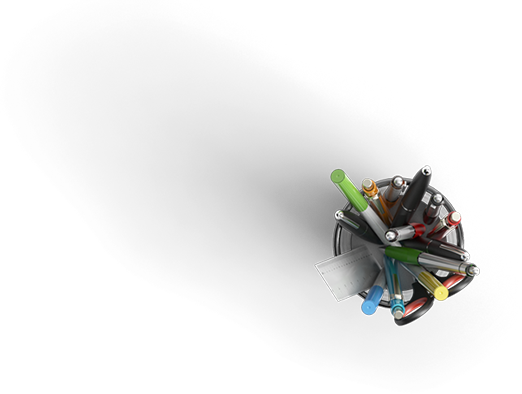



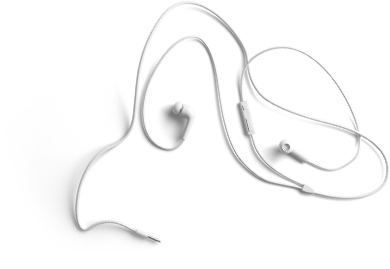
Evaluation of Machine Learning Algorithms for Classifying Deep Brain Stimulation Respective of On and Off Status
Mar 17, 2020 | Medical
Our Service Charter
1. Professional & Expert Writers: Topnotch Essay only hires the best. Our writers are specially selected and recruited, after which they undergo further training to perfect their skills for specialization purposes. Moreover, our writers are holders of masters and Ph.D. degrees. They have impressive academic records, besides being native English speakers.
2. Top Quality Papers: Our customers are always guaranteed of papers that exceed their expectations. All our writers have +5 years of experience. This implies that all papers are written by individuals who are experts in their fields. In addition, the quality team reviews all the papers before sending them to the customers.
3. Plagiarism-Free Papers: All papers provided by Topnotch Essay are written from scratch. Appropriate referencing and citation of key information are followed. Plagiarism checkers are used by the Quality assurance team and our editors just to double-check that there are no instances of plagiarism.
4. Timely Delivery: Time wasted is equivalent to a failed dedication and commitment. Topnotch Essay is known for timely delivery of any pending customer orders. Customers are well informed of the progress of their papers to ensure they keep track of what the writer is providing before the final draft is sent for grading.
5. Affordable Prices: Our prices are fairly structured to fit in all groups. Any customer willing to place their assignments with us can do so at very affordable prices. In addition, our customers enjoy regular discounts and bonuses.
6. 24/7 Customer Support: At Topnotch Essay, we have put in place a team of experts who answer to all customer inquiries promptly. The best part is the ever-availability of the team. Customers can make inquiries anytime.
Recent Posts
- Positive Psy-5.1 Discerning Truth Getting Started
- Given your understanding of the functions of the different lobes of the brain, how might a person be affected by damage to the temporal lobe?
- History Discussion Assignment
- Discussion: Explore how lifestyle changes-such as nutrition–can prevent or modify disease processes
- Read the article on Spirit Airlines
Archives
- January 2025
- August 2024
- July 2024
- June 2024
- May 2024
- April 2024
- January 2024
- December 2023
- August 2023
- July 2023
- June 2023
- May 2023
- January 2023
- October 2022
- September 2022
- August 2022
- July 2022
- June 2022
- May 2022
- March 2022
- February 2022
- January 2022
- December 2021
- November 2021
- October 2021
- September 2021
- August 2021
- July 2021
- June 2021
- May 2021
- April 2021
- March 2021
- February 2021
- January 2021
- December 2020
- November 2020
- October 2020
- September 2020
- August 2020
- July 2020
- June 2020
- May 2020
- April 2020
- March 2020
- February 2020
- January 2020
- December 2019
- November 2019
- October 2019
- September 2019
Categories
- `
- .uul l illl'
- "" ""Qualitative Analysis Assignment: Transcript 3
- "" which is attached to this assignment.
- (15 points) Lot Ai? ?Xn be a random sample from a f/(0,0) population with unknown parameter 0 > 0. Find the likelihood ratio test (LRT) of size a for testing Ho : 0 > 0q .vs. H : 0 < Oq.
- { ""cell_type"": ""markdown""
- /files/-np-ex19-7b-xlsx
- /files/0886109913504154-pdf,/files/1363460706065057-pdf,/files/0886109914560741-pdf
- /files/11357-txt,/files/cavell-pdf
- /files/20191118150623part-vii-assessment-tool-development-rub1-pdf
- /files/20191119051422iom-report-future-of-nursing-rubric-xlsx
- /files/20200428035024defend-or-refute-guide-pdf
- /files/adjustmentdisorder-pdf,/files/depression1-pdf-6973733
- /files/assignment1j-docx-7815535,/files/assignment2j-docx
- /files/assignment2-docx-5634861,/files/networking14-pkt,/files/20200204-routers-table-docx,/files/20200204-network-infrastructure-design-pdf-5640375
- /files/assignment2-docx-8830717
- /files/bookaccess-docx-5088805
- /files/bookchap1-412-pdf,/files/bookchap1-412-pdf-7977187
- /files/businessculturaldimensionsanalysisgradingrubric-pdf-7789673"
- /files/casestudy2-docx-5603093
- /files/chapter5-pdf-7822037
- /files/crjs3001wk1studentreplies-docx
- /files/developingobjectives1-docx,/files/article3assignments3-41-pdf,/files/developingobjectives1-docx-7919359
- /files/discussionreflectionguide-docx,/files/introduction-to-criminology-10th-edition-9781544339023-pdf,/files/sample-docx-7598097
- /files/essay2-documentedargumentrevised22-pdf-6947741
- /files/frenchandindianwar-docx-7926377,/files/bostonteapartyaftermath-docx
- /files/homework2negotiationmorgan-docx,/files/homeworknegotiation1michele-docx
- /files/labassignmnetw8-docx-7665133
- /files/lessonweek4-docx
- /files/mat240houselistingpricebyregionpjct5-xlsx-7794861,/files/mat240modulefiveassignmenttemplatepjct5-docx-7794863
- /files/microm53question-docx
- /files/milecture-pdf,/files/motivationalinterviewing-thebmj-pdf
- /files/nrnpprac6635comprehensivepsychiatricevaluationtemplate-docx-8263023"
- /files/principlesofcorporatefinance13erichardbrealey-pdf-7629311,/files/wk71-docx,/files/wk72-docx,/files/wk73-docx,/files/wk74-docx
- /files/problemstatementchecklist-pdf-6967979,/files/week4article-pdf-6967981
- /files/prospectusfinaldraft-correctedversion-docx,/files/dissertationprospectusoriginalversion-docx
- /files/reflectionpaperguidelines-docx-7570139
- /files/rubricpandv-pdf-7584061,/files/page11-pdf-7584063,/files/page9-10-pdf-7584065,/files/implicationofpiagetsandvygotskytheories-summer2021socialstudiesmethodsforece-fieldece-3571-01-pdf
- /files/screenshot2021-02-27at11-35-38am-png"
- /files/sec-516-t6-meetingtheneedsofdiverselearners-docx"
- /files/spd-400-d-t6-studentgoalsandinterventionplantemplate-docx-7938583,/files/rub-docx-7938623
- /files/taskdifficultyandincubation1-docx
- /files/unitvinternationlstudyguide-pdf-7796117
- /files/visualcommunicationassessment23051-docx
- /files/week3assignmentg-docx
- /files/week4rubric-docx-7856191
- /files/week7readingdestructivebehavior-pdf-7890761,/files/bibliu-print-9781452236315table143-pdf
- /files/westernphilosophyananthologybycottinghamjohnz-lib-org-pdf"
- /files/woodch9-pdf,/files/chapter8-pdf-6111751
- /files/youthexposedtoviolenceindc-august2021-docx,/files/chapteronethenatureandtoolsofresearch-pdf
- ~I'vcrn I""theorems"" follow from it
- 1111 writing and reading is embedded in some Discourse
- 12 font
- 12 point font
- 2015
- 2021
- 2021). HTML pages are an excellent example that allows network shares hence makes the embedding of the resources possible. Therefore
- 3 PAGE ESSAY ON WHY I DONT WANT ANY TATTOOS. I NEED CLEAR EXAMPLES
- 4-6sentence · Sentence1-2:Author + Genre + Context of the Bible book (that the story is a part of) · Sentences 3-5: Brief Summary Including Story Genre (historical narrative, mythology, or parable).
- 4)."
- Acc 206
- ACC 206, BUSINESS FINANCE
- ACC201
- ACC544, BUSINESS FINANCE
- ACC556
- ACC573, Business & Finance
- ACC60171, Other
- Accounting & Finance
- Acct 101
- ACCTG406
- ACIS5104
- ACOL202
- acquire (at least) one initial Discourse. This initial Discourse
- actively engaging with course materials
- acts
- AD712, Business & Finance
- adding beliefs
- ADMIN565
- ADMN575, OTHER
- allocating specific time slots for coursework
- ALY6100
- American Military University
- AMERICAN MILITARY UNIVERSITY, BUSINESS FINANCE
- AMERICAN MILITARY UNIVERSITY, WRITING
- American Public University System
- AMERICAN PUBLIC UNIVERSITY SYSTEM, BUSINESS FINANCE
- AMERICAN PUBLIC UNIVERSITY SYSTEM, HUMANITIES
- AMH2020
- analyze and evaluate the following questions:
- and cost were controlled.
- and academic grammar and usage.
- and architecture the guys work execution at the program or system level. At the risk of falling victim to stating the obvious
- and describe the type of economic analysis that you would use in the evaluation.
- and external resources recommended by instructors. Utilize online libraries
- and interactive components. Take comprehensive notes
- and managing your time effectively
- and often write
- and personal health record (PHR)
- and related terms for two separate concepts. (examples: technology-computer
- and Transportation and Telecommunication. Using The World Factbook
- and users are able to inject SQL commands using the available input (Imperva
- ANM104
- ANM104 OL1
- ANTH130, SCIENCE
- ANTHROP 2200
- Anthropology 130
- Applied Science
- Applied Sciences
- Applied SciencesApplied Sciences
- Architecture and Design
- Architecture and DesignArchitecture and Design
- Arizona State University
- ARIZONA STATE UNIVERSITY, WRITING
- Art
- ART101
- ART2010
- ARTH102
- article
- Arts
- ARTS1301
- ASC400, BUSINESS FINANCE
- ashford university
- ASHFORD UNIVERSITY, BUSINESS FINANCE
- ASHFORD UNIVERSITY, HUMANITIES
- ASHFORD UNIVERSITY, OTHER
- ASHFORD UNIVERSITY, SCIENCE
- Ashworth College
- asking thoughtful questions and providing constructive feedback to your peers. Regularly check your course emails and notifications
- assignments
- at least
- Atlantic International University Online, Science
- attitudes
- BADM735
- BAM515, BUSINESS FINANCE
- BCJ3601, BUSINESS FINANCE
- BCN4431
- BEHS380, WRITING
- Berkshire Community College
- Bethel University
- BETHEL UNIVERSITY, HUMANITIES
- beyond the family and immediate kin and peer group. These may be 1111.
- BHR3352
- BHR3352 Human Resource Management
- BIO1100
- BIO1408
- BIO2401
- BIO3320
- BIO354, SCIENCE
- BIOCHEM202
- Biology
- Biology – Anatomy
- Biology – AnatomyBiology – Anatomy
- Biology – Ecology
- Biology – Physiology
- BIOLOGY 10, SCIENCE
- BiologyBiology
- Blog
- BME351
- body
- Bowie State University
- Bowie State University, Science
- brings with it the (poten- u.il) acquisition of social ""goods"" (money
- BROCK UNIVERSITY, BUSINESS FINANCE
- BROCK UNIVERSITY, OTHER
- BROOKDALE COMMUNITY COLLEGE, HUMANITIES
- BROOKLYN COLLEGE, WRITING
- BUAD326, Business & Finance
- BULE303
- BUS1001
- BUS120, Business & Finance
- BUS125, WRITING
- BUS187, Business & Finance
- BUS232
- BUS242
- BUS303, BUSINESS FINANCE
- BUS410, BUSINESS FINANCE
- BUS472, SCIENCE
- BUS475
- BUS499
- BUS520, Business & Finance
- BUS530
- BUS542
- BUS599
- BUS620
- BUS623
- BUS630
- BUSI320
- Business
- Business – Management
- Business & Finance
- Business & Finance – Financial markets
- Business & Finance – Financial marketsBusiness & Finance – Financial markets
- Business & Finance – Marketing
- Business & Finance – MarketingBusiness & Finance – Marketing
- Business & Finance – Supply Chain Management
- Business & Finance , BUS430
- Business & Finance , BUSN370
- Business & Finance , COMM240
- Business & Finance , COMS2302
- Business & Finance , ENT527
- Business & Finance , FIRE3301
- Business & Finance , G141COM1002
- Business & Finance , GB520
- Business & Finance , GB540
- Business & Finance , IBSU487
- Business & Finance , JWI515 Managerial Economics
- Business & Finance , MGT16
- Business & Finance , MGT496
- Business & Finance , MGT498
- Business & Finance , MGT521
- Business & Finance , MT460
- Business & Finance , PM586
- Business & Finance , RMI3348
- Business & Finance , SOC450
- Business & Finance , south university online
- Business & Finance , Strayer University
- Business & Finance , University of Phoenix
- Business & Finance , Wilmington University
- Business & Finance, Trident University
- Business & FinanceBusiness & Finance
- Business and Finance
- Business Finance – Accounting
- Business Finance – AccountingBusiness Finance – Accounting
- Business Finance – Economics
- Business Finance – EconomicsBusiness Finance – Economics
- Business Finance – Management
- Business Finance – ManagementBusiness Finance – Management
- Business Finance – Operations Management
- Business Finance – Operations ManagementBusiness Finance – Operations Management
- BUSINESS FINANCE, CBBU1001
- BUSINESS FINANCE, COLORADO STATE UNIVERSITY GLOBAL
- BUSINESS FINANCE, COLORADO TECHNICAL UNIVERSITY
- BUSINESS FINANCE, COLUMBIA SOUTHERN UNIVERSITY
- BUSINESS FINANCE, COM 510
- BUSINESS FINANCE, CRJ101
- BUSINESS FINANCE, DOC660
- BUSINESS FINANCE, EASTERN KENTUCKY UNIVERSITY
- BUSINESS FINANCE, ECN 501
- BUSINESS FINANCE, ECO2251
- BUSINESS FINANCE, ECO531
- BUSINESS FINANCE, FIN 500
- BUSINESS FINANCE, FIN31FMS12019
- BUSINESS FINANCE, GRANTHAM UNIVERSITY
- BUSINESS FINANCE, HLS3302
- BUSINESS FINANCE, HRC164
- BUSINESS FINANCE, HRM 500
- BUSINESS FINANCE, INDS 400
- BUSINESS FINANCE, INT113
- BUSINESS FINANCE, INTL3306
- BUSINESS FINANCE, ISDS 351
- BUSINESS FINANCE, LAWS OF EVIDENCE
- BUSINESS FINANCE, LIBERTY UNIVERSITY
- BUSINESS FINANCE, MAN3504
- BUSINESS FINANCE, MBA 5121
- BUSINESS FINANCE, MG260
- BUSINESS FINANCE, MGMT386
- BUSINESS FINANCE, MGT 521
- BUSINESS FINANCE, MGT211
- BUSINESS FINANCE, MICHIGAN STATE UNIVERSITY
- BUSINESS FINANCE, MIDDLE TENNESSEE STATE UNIVERSITY
- BUSINESS FINANCE, MKT331
- BUSINESS FINANCE, MKT419
- BUSINESS FINANCE, NORTHEAST MONTESSORI INSTITUTE
- BUSINESS FINANCE, OAKLAND UNIVERSITY
- BUSINESS FINANCE, PARK UNIVERSITY
- BUSINESS FINANCE, RASMUSSEN COLLEGE
- BUSINESS FINANCE, SOUTHERN NEW HAMPSHIRE UNIVERSITY
- BUSINESS FINANCE, TRIDENT INTERNATIONAL UNIVERSITY
- Business Strategy
- C11E
- Calculus
- California Baptist University
- California Coast University
- CALIFORNIA STATE UNIVERSITY NORTHRIDGE, OTHER
- can be studied
- can never 21 really be liberating literacies. For a literacy to be liberating it must contain both the Discourse it is going to critique and a set of meta-elements (language
- Capella University
- Capella University, Humanities
- Capital L. George Adams
- CATEGORY
- CE304
- CE445
- CEE792
- CEGR338
- Chamberlain College of Nursing
- Chapter 3
- Charles R Drew University of Medicine and Science
- charles sturt university
- Chaudhary Charan Singh University, Humanities
- CHEM101
- CHEM111
- CHEM1411, Science
- CHEM202, Science
- CHEM210, Science
- CHEM410
- Chemistry
- Chemistry – Chemical Engineering
- Chemistry – Organic chemistry
- Chemistry – Pharmacology
- Chemistry – Physical chemistry
- ChemistryChemistry
- Childcare
- CHMY373, SCIENCE
- Choose three problematic issues that are currently facing older people living in the community?
- CINE286U
- CIS210
- cis273
- CIS359
- CIS510, Other
- CIS524
- CIVL6603, Science
- CJ430, SCIENCE
- CJA444
- CJUS300, Other
- Classics
- CMIT495
- CMSC140
- Colorado Christian University
- COLORADO STATE UNIVERSITY GLOBAL, SCIENCE
- COLORADO STATE UNIVERSITY, SCIENCE
- Colorado State UniversityGlobal
- Colorado Technical University
- COLORADO TECHNICAL UNIVERSITY, OTHER
- Colorado Technical University, Programming
- Columbia Southern University
- COLUMBIA SOUTHERN UNIVERSITY, OTHER
- Columbia Southern University, Science
- COLUMBIA SOUTHERN UNIVERSITY, WRITING
- Commerce
- Communication
- Communications
- COMMUNITY-BASED CORRECTIONS
- COMP1007
- Computer Science
- Computer Science – Java
- Computer Science- Python
- Computer ScienceComputer Science
- Construction
- correctness
- Cosc1437
- counseling chemical dependency adolescents
- Criminology
- CRJ305
- cross-site scripting
- CS101
- CSIT 100, PROGRAMMING
- CSPM326
- CST 610, PROGRAMMING
- Cultural Studies
- culturally appropriate intervention to address childhood obesity in a low-income African American community.
- CUR535
- CUYAMACA COLLEGE, HUMANITIES
- CUYMACA COLLAGE, HUMANITIES
- Data Analysis
- DAVIDSON COLLEGE, OTHER
- defensive programming allows for more efficient processes while also protecting systems from attack.
- DES201
- Describe the difference between glycogenesis and glycogenin ? Explain in 10 to 12 lines.
- Design
- Digital Marketing Plan for Nissan Motor Co. The plan will identify the current marketing opportunity and/or problem(s) and propose digital marketing solutions. Please use header in the attachment."
- Discuss one way in which the Soviet Union fulfilled communist thought, and another way in which it did not with reference to O'Neil's Chapter 9.
- Discuss the pros and cons of free-market based economies and how they impact the modern, globalized economy? What comes to your mind when you hear the term "globalization?"
- Dissertation
- DMM612, Science
- DMM649, SCIENCE
- Draft and essay of 1,000 words minimum, stating the Most Important and Relevant aspects to be considered when carrying on INTERNATIONAL NEGOTIATIONS or MULTI-CULTURAL NEGOTIATIONS.
- Drama
- each time a user extracts the ZIP file
- Earth Science – Geography
- Earth Science – GeographyEarth Science – Geography
- Earth Science – Geology
- EAS1601
- ECD 541, HUMANITIES
- ECE 452
- Ecommerce
- ECON335
- Economics
- ECPI University
- EDUC696
- Education
- EducationEducation
- EEL3472C
- EEL3705
- EET110
- EFFAT UNIVERSITY JEDDAH, HUMANITIES
- elasticity
- ELI2055A
- EMDG 230, SCIENCE
- Emglish
- Emory University
- Employment
- EN106
- EN106, HUMANITIES
- EN109
- EN206, HUMANITIES
- ENC1102, Writing
- eng 100
- ENG100
- ENG101
- ENG101, Humanities
- ENG102
- ENG102, Humanities
- eng106
- ENG1102, WRITING
- ENG124
- ENG124, Humanities
- ENG124, Writing
- ENG1340, HUMANITIES
- ENG200, Humanities
- ENG207
- eng2206
- ENG2211
- ENG305
- ENG812
- Engineering
- Engineering – Chemical Engineering
- Engineering – Civil Engineering
- Engineering – Civil EngineeringEngineering – Civil Engineering
- Engineering – Electrical Engineering
- Engineering – Electrical EngineeringEngineering – Electrical Engineering
- Engineering – Electronic Engineering
- Engineering – Mechanical Engineering
- Engineering – Mechanical EngineeringEngineering – Mechanical Engineering
- Engineering – Telecommunications Engineering
- EngineeringEngineering
- ENGL 120
- ENGL 124, OTHER
- ENGL 124, WRITING
- ENGL 2030, HUMANITIES
- ENGL1102
- ENGL120, HUMANITIES
- ENGL120SP2019, WRITING
- ENGL126
- ENGL1302
- ENGL130E, HUMANITIES
- ENGL147N, HUMANITIES
- ENGL2, Humanities
- English
- English – Article writing
- English – Article writingEnglish – Article writing
- English Language
- English Literature
- EnglishEnglish
- ENGR350
- ENST202CORE274
- ensuring you allocate dedicated time for coursework
- Environment
- Environmental Science
- Environmental Sciences
- Environmental Studies
- especially for a small company
- Essay Writing
- etc¦). Please note at least five organizational activities and be specific when responding.
- ETH321
- ETHC445N
- Ethnic Studies 101
- European Studies
- EXNS6223
- Family
- Fashion
- February 20). What is defensive programming? EasyTechJunkie. Retrieved December 30
- film industry
- FILM INDUSTRY, HUMANITIES
- Film Studies
- FIN 500
- FIN330, MATHEMATICS
- FIN370
- Final Essay
- Find the uniform most powerful level of alpha test and determine sample size with the central limit theorem
- Florida International University
- Florida National University
- Florida State College at Jacksonville
- FoothillDe Anza Community College District
- Foreign Languages
- Foreign Languages – Spanish
- formulations
- from https://www.pcmag.com/news/fat32-vs-ntfs-choose-your-own-format
- G124/enc1101
- Gallaudet University
- General Studies
- General_Business
- GEO1206
- GEOG100, Science
- Geography
- GEOL3200, HUMANITIES
- Geometry
- George Mason University
- GERM1027
- GERO 101, SCIENCE
- GERON101
- GLG101, Science
- GO16
- Government
- GovernmentGovernment
- GOVT2305
- GOVT2305, Humanities
- GOVT2306
- Grand Canyon University, Science
- Grand Canyon University, Writing
- Grantham University
- GRANTHAM UNIVERSITY, PROGRAMMING
- GRANTHAM UNIVERSITY, WRITING
- GROSSMONT COLLEGE, HUMANITIES
- Grossmont-Cuyamaca Community College District
- GROSSMONT-CUYAMACA COMMUNITY COLLEGE DISTRICT, HUMANITIES
- GU299, WRITING
- Hawaii Pacific University
- HC310
- HCA415
- HCA521
- HCM550, SCIENCE
- Hcs370
- HCS446
- he focused on aspects of the U.S. that combined democratic and increasingly capitalist characteristics. THINK ABOUT the points De Tocqueville made.
- Health & Medical
- Healthcare
- HIM 2588, MATHEMATICS
- HIM 500, SCIENCE
- HIM301
- HIS 108
- HIS101
- HIS105
- HIS200
- HIST104A, Humanities
- HIST111
- HIST1301, HUMANITIES
- HIST1302
- HIST1320
- HIST1700
- HIST2620
- HIST350, Humanities
- HIST405N, HUMANITIES
- HIST459, Humanities
- History
- History – American history
- History – American historyHistory – American history
- History – Ancient history
- History – Ancient historyHistory – Ancient history
- History – World history
- History – World historyHistory – World history
- HISTORY4250, Humanities
- HistoryHistory
- HLSS508, OTHER
- HMP403
- Hospitality
- HOST1066, WRITING
- Housing
- How do the changes in ship technology effect port operations? Discuss at least 3 factors contributing to port operations and development. Address cargo and passenger liners.250 words
- How have Mary Calderone, SIECUS and other sex educators changed how sex education is perceived? (100 words minimum)
- HOWARD UNIVERSITY, SCIENCE
- HR Management
- HRM300
- HRT6050, Writing
- HSA305
- HSA535
- HSC3201
- HSN476
- HUM1002
- HUM115
- HUM115, Writing
- Human Resource
- Human Resource Management
- Human Resource ManagementHuman Resource Management
- Human Resources
- HUMAN RESOURCES DEVELOPMENT AND MANAGEMENT, SCIENCE
- Human Rights
- HUMANITIES
- Humanities, Alcorn State University
- HUMANITIES, HY 1110
- Humanities, LMC3225D
- HUMANITIES, LONG BEACH CITY COLLEGE
- HUMANITIES, MUSIC1306
- HUMANITIES, OAKLAND COMMUNITY COLLEGE
- HUMANITIES, PH 100
- HUMANITIES, POINT LOMA NAZARENE UNIVERSITY
- HUMANITIES, PRINCE GEORGE'S COMMUNITY COLLEGE
- Humanities, PSY105
- HUMANITIES, PSY330 THEORIES OF PERSONALITY
- Humanities, PSYC 1101
- HUMANITIES, PSYCH305
- HUMANITIES, PSYCH635 PSYCHOLOGY OF LEARNING
- HUMANITIES, RSCH8110
- HUMANITIES, SAN DIEGO STATE UNIVERSITY
- HUMANITIES, SAN JACINTO COLLEGE
- Humanities, SOC1010
- HUMANITIES, SOC401
- HUMANITIES, SOCIOLOGY OF RELIGION
- HUMANITIES, SOUTHERN NEW HAMPSHIRE UNIVERSITY
- HUMANITIES, STRAYER UNIVERSITY
- HUMANITIES, SWK110
- HUMANITIES, UNIVERSITY OF CALIFORNIA
- HUMANITIES, UNIVERSITY OF CALIFORNIA IRVINE
- HUMANITIES, UNIVERSITY OF HOUSTON-DOWNTOWN
- Humanities, University of Maryland University College
- i need the attached work to look like this. please redo and make it look like this.
- I need these questions answered fully. I have the assignment and the notes attached for it. Do not use chegg or course hero. This is due Wednesday 4/14 at 10:00 pm which is almost 4 full days. Thanks!
- I need to re organize a research paper I attached all my information and I attached you an example how is going to be. Please follow the instruction and the references has to be APA 7edition
- Identify a cardiac or respiratory issue and outline the key steps necessary to include for prevention and health promotion
- identify the leadership theory that best aligns with your personal leadership style
- if you suggest trying to do this
- IGLOBAL UNIVERSITY
- IHS2215
- Iii Mlch
- III nuistery of such superficialities was meant to
- Implement classifiers KMeans, Random Forest and Decision Tree, SVM,XGBoost and Naive Bayes for the given dataset of audio samples to findout top genre for an audio sample(which one fits best)
- In a cardiac issue what are the key steps necessary to include for prevention and health promotion.
- in any other way
- include a paragraph about which side of the case a forensic psychologists might support and why.
- indeed
- India
- INDIANA UNIVERSITY BLOOMINGTON, SCIENCE
- INF690
- INF690, Other
- Information Systems
- Information SystemsInformation Systems
- Information Technology
- INSTITUTE OF PUBLIC ADMINISTRATION SAUDI ARABIA, PROGRAMMING
- INT700, OTHER
- International Business
- International Relations
- International Studies
- Internet
- Introduction to Biology
- Is jury nullification sometimes justifiable? When?
- ISSC351
- It Research
- IT380
- IT550, Business & Finance , Southern New Hampshire University
- ITC3001
- ITP120
- ITS 631, PROGRAMMING
- ITS835, Other
- JEDDAH COLLEGE OF ADVERTISING, WRITING
- Journalism
- KNOWLEDGE IS POWER, OTHER
- Languages
- Law
- Law – Civil
- Law – CivilLaw – Civil
- Law – Criminal
- Law – CriminalLaw – Criminal
- LawLaw
- Leadership
- lecture slides
- Leisure Management
- Liberty University
- LIBERTY UNIVERSITY, WRITING
- lIlgll.Igt· (1II1In·d
- Linguistics
- literacy is always plural: literacies (there are many of them
- Literature
- Literature Review
- Literature review funnel on "cyber security"
- LiteratureLiterature
- MA105
- MAJAN COLLEGE, WRITING
- Management
- Manpower
- Marketing
- Math
- MATH 1030
- MATH144, MATHEMATICS
- Mathematics
- Mathematics – Algebra
- Mathematics – Calculus
- Mathematics – Geometry
- Mathematics – Numerical analysis
- Mathematics – Precalculus
- Mathematics – Probability
- Mathematics – Statistics
- Mathematics – StatisticsMathematics – Statistics
- Mathematics – Trigonometry
- MATHEMATICS, MGT3332
- Mathematics, National American University
- Mathematics, PSY325
- MATHEMATICS, PUBH8545
- Mathematics, QNT275
- MATHEMATICS, STAT 201
- MBA503
- McMaster University
- ME350B, SCIENCE
- MECH4430, SCIENCE
- Mechanics
- Media
- Medical
- Medical Essays
- MGMT2702
- MGMT410
- MGT173, SCIENCE
- MHR6451
- MIAMI UNIVERSITY, WRITING
- Military
- Military Science
- MKT501
- MKT690, OTHER
- MN576
- MN581
- MN610, SCIENCE
- MNGT3711
- Music
- MVC109
- N4685
- NATIONAL INSTITUTES OF HEALTH, SCIENCE
- NATIONAL UNIVERSITY, SCIENCE
- Needs to be at least 300 wordswithin the past five years.No plagiarism! What key aspects do you believe should guide ethical behavior related to health information, technology, and social media?
- no workable ""affirmative action"" for Discourses: you can't 19 Ill' let into the game after missing the apprenticeship and be expected to have a fnir shot at playing it. Social groups will not
- Northcentral University
- not writing)
- nothing can stand in her way once she has her mind set. I will say that she can sometimes be hard headed
- Nova Southeastern University
- NR447, SCIENCE
- NRS429VN
- NRS44V, OTHER
- NRS451VN
- NRSE4540
- NSG426
- NSG486
- NSG6102
- NSG6102, SCIENCE
- Numerical Analysis
- NUR231NUR2349, SCIENCE
- NUR647E
- NURS350
- NURS508
- NURS6640
- Nursing
- NURSING LEADERSHIP AND MANAGEMENT, SCIENCE
- NursingNursing
- Nutrition
- offering learners the flexibility to acquire new skills and knowledge from the comfort of their homes. However
- OHIO UNIVERSITY, SCIENCE
- Online Discussion Forums Grade and Reflection Assignment : Current Topic Artificial Intelligence HR Planning Career and Management Development Labour RelationsForum
- operation security
- Operations Management
- or do those companies have an ethical obligation to protect people? In this assignment
- ORG5800, OTHER
- Organisations
- OTHER
- Other, PAD631
- OTHER, PARK UNIVERSITY
- OTHER, PLA1223
- Other, POLI330N
- OTHER, PROFESSIONAL NURSING NU231 NUR2349
- Other, RTM404
- OTHER, SAINT LEO UNIVERSITY
- OTHER, SOC3210C1
- Other, SOCW6333
- OTHER, SOUTHERN NEW HAMPSHIRE UNIVERSITY
- Other, The University Of Southern Mississippi
- OTHER, TRIDENT UNIVERSITY INTERNATIONAL
- Other, UC
- OTHER, UNIVERSITY OF MARYLAND UNIVERSITY COLLEGE
- OTHER, UNIVERSITY OF SOUTH FLORIDA
- Other, Walden University
- paying attention to grammar and spelling. Actively participate in discussions
- Personal Development
- PhD Dissertation Research
- PHI 413V, SCIENCE
- Philosophy
- Photography
- PHY290
- PHYS204L206
- Physics
- Physics – Astronomy
- Physics – Electromagnetism
- Physics – Geophysics
- Physics – Mechanics
- Physics – Optics
- PhysicsPhysics
- Physiology
- PNGE332, SCIENCE
- Political Science
- Political SciencePolitical Science
- Politics
- PowerPoint slides
- privacy
- PROFESSIONAL NURSING NU231 NUR2349, SCIENCE
- PROFESSIONAL NURSING NU231NUR2349, SCIENCE
- Programming
- Programming , College of Applied Sciences
- PROGRAMMING, STRAYER UNIVERSITY
- PROGRAMMING, WILMINGTON UNIVERSITY
- Project Management
- proper grammar
- Protein
- provide a discussion on what could have been done better to minimize the risk of failure. If you have not yet been involved with a business process redesign
- PSYC8754, WRITING
- Psychology
- PsychologyPsychology
- PUB373, SCIENCE
- Purdue University
- Rasmussen College
- Read a poam and write a paragraph to prove "The table turned".
- Reading
- ReadingReading
- readings
- Reference this
- REL1030
- Religion
- RES861, Science RES861
- Research Methodology
- Research methods
- Research Proposal
- Research questions
- Retail
- Rutgers university
- SAFE4150
- safety statutes
- Santa Clara University
- SCI 220, SCIENCE
- SCI115, SCIENCE
- Science
- Science, Strayer University
- SCIENCE, THOMAS JEFFERSON UNIVERSITY
- SCIENCE, WEST COAST UNIVERSITY
- SCIENCE, WEST TEXAS A & M UNIVERSITY
- Sciences
- SCM371, Writing
- Search in scholarly sources the similarities and difference between PhD and DNP. Post three similarities and three differences found on your research. Do not forget to include your reference.
- Security
- self-actualization
- several things can happen
- Should the government operate public transportation systems?250 words
- so that it is not biased?
- so too
- SOC 450
- Social Policy
- Social Science
- Social Science – Philosophy
- Social Science – PhilosophySocial Science – Philosophy
- Social Science – Sociology
- Social Science – SociologySocial Science – Sociology
- Social Sciences
- Social ScienceSocial Science
- Social Work
- Society
- Sociology
- someone cannot engage in a Discourse in a less than fully fluent manner. You are either in it or you're not. Discourses are connected with displays of
- SP19, WRITING
- SPC2608
- SPD310
- Sports
- Statistics
- succeeding in online courses requires a different approach compared to traditional classroom settings. To help you make the most of your online learning experience
- such as notifications from social media or email. Organize your study materials and have a reliable internet connection to ensure seamless access to course materials.
- Technology
- that personal ethics and organizations ethics are two different and unrelated concepts. Others
- the attribute is useful
- The directions are attached. However you must read the PDF file first in order to answer the questions.
- the role of work and money
- Theatre
- then reply to a minimum of 2 of your classmates' original posts.
- Theology
- Threat of artificial intelligence 800 words.
- to be true of second language acquisition or socially situ ated cognition (Beebe
- to better promote the value and dignity of individuals or groups and to serve others in ways that promote human flourishing.
- to usc a Discourse. The most you can do is III It'! them practice being a linguist with you.
- total fat consumption
- Tourism
- Translation
- Transportation
- U110
- Uncategorized
- University of Central Missouri
- University of South Florida
- UNIVERSITY OF SOUTH FLORIDA, WRITING
- Video Games
- Watch this meditation https://www.youtube.com/watch?v=doQGx4hdF3M&feature=youtu.be and write a one page reflection
- WCWP10B
- we can always ask about how much ten- 12 """""" or conflict is present between any two of a person's Discourses (Rosaldo
- What approaches to the study of poverty does economic sociology offer? More specifically, what might sociologists studying poverty focus on besides poor households, neighborhoods, and individuals?
- What is the philosophical matrices for Behaviorism, Constructivism, and Reconstructivism
- What key aspects do you believe should guide ethical behavior related to health information, technology, and social media?
- what place did government have to improve markets? What does the concept of ""crowding out"" mean in all of this?
- What should be done to maintain optimum stock levels and why is it important to keep accurate and up-to-date records of stock in medical practice?
- whether good or bad. The intent of the short research projects is to dig a little deeper into some of the topics
- which triggers the vulnerability. As soon as the user downloads this shortcut file on Windows 10; windows explorer will
- Would somebody read these quotes and answer those three questions at the bottom for me?Disregard number two I will look for myself in the text.
- Write short essay based on evidence about the 2 cons of Sex Education 250-300 words 2 reference minimum no plagiarism
- WRITING
- writing assignment, you will analyze asymmetric and symmetric encryption. Evaluate the differences between the two of them and which one that you would determine is the most secure.
- Writing, Personal Code of Technology Ethics
- you believe you can provide the CIO with the information he needs.
- you will learn how to search for scholarly
- you will need to read the TCP standard. TCP was first defined in RFC 793. A link to this document is provided. https://tools.ietf.org/html/rfc793
- Young People
Recent Comments