COMPARATIVE ANALYSIS OF MACHINE LEARNING ALGORITHMS IN SPEAKER RECOGNITION OF WHISPERED SPEECHABSTRACTSpeaker recognition is the procedure of naturally perceiving who is speaking by utilizing speaker-specific data incorporated into the speech waves. The speaker-specific data act as the unique characteristics of the speakers voices. The objective of speaker recognition is to extract, characterize and recognize the information about the speakers identity. This has risen as one of the critical application region of Speech and Language Processing. This work proposes an investigation of different algorithmic methods: Support Vector Machines (SVM), K-Means Clustering, Gradient Boosting, EXTRA Trees and Random Forests for the recognition of the speaker of the whispered discourse. The whisper sounds from the CHAIN Whispered Speech corpus are utilized in the trials. Feature vectors from the corpus are extracted by utilizing Mel-Frequency Cepstral Coefficients (MFCC) which convey the speakers voice attributes. These coefficients are then used to recognize the obscure speaker from a given arrangement of speakers by implementing the algorithms.INTRODUCTIONSpeaker Recognition involves the identification of the exact person who is speaking. This specific technology can be used in various facets associated with our lives for identification confirmation in biometrics. Biometrics handle the process associated with authentication using unique features of the person. There are usually numerous strategies for biometrics like irises, fingerprints, talk, and face [1]. Many administrations incorporate voice dialing, banking transactions, database administrations, voice message and security control for classified data, remote access to systems and as a forensics tool. As all these strategies are unique to every single individual, they cannot be replicated, copied or stolen. Hence, among the modalities, speech is advantageous as it is easily obtainable and enables convenient usability. Thus biometrics universally offer more protected and forthcoming means associated with identity authentication. Rather than regular speech, this paper focuses on whispered speech. Whispered speech allows high privacy intended in certain tasks to ensure security. Whispered speech differs from normal speech due to its low sound pressure level and lack of pitch frequency. In contrast to natural speech, whispered speech has a different short-term amplitude distribution. Since whispered speech has low Signal Noise Ratio (SNR), it becomes difficult to be replicated thus preventing invasion in a security system. The speaker is initially unknown, and must be determined after being compared to the templates trained. There is often a very large number of templates that are involved in identifying a speaker. Thus, precise identification of the speaker is a difficult task and hence, speaker recognition becomes an active research area. Speaker Recognition works on the premise that a persons speech exhibits characteristics that are unique to the speaker. However this task is questioned by the possible variants of input speech signals [2].During the course of development in speaker recognition, there have been a number of researches presented in literature. Several recent approaches using support vector machines have been proposed for speech applications. The first set of approaches attempts to model emission probabilities for hidden Markov models [3], [4]. Even though this approach reduces error to an extent, this method still has problems. Firstly, there is an excessive need for large training sets result in long training times for support vector methods. The emission probabilities must be approximated [5], since the output of the support vector machine is not a probability. Another model for speaker identification or verification presents work using a subspace-based enhancement technique and probabilistic support vector machines (SVMs) [6], [7]. A perceptual filter-bank is created. The prior Signal to Noise Ratio (SNR) of each sub-band within the perceptual filter-bank, which is used to decide the estimators gain, to effectively suppress background noises. A probabilistic Support Vector Machine is then used to identify or verify the speaker. Major drawback faced by these models is that searching for a suitable hyperplane in input space, which is extremely restrictive to be of practical use.The Gaussian Mixture Model (GMM) [8], [9] have become a dominant approach for robust text-independent speaker identification. In [8], the individual Gaussian components of a GMMs are shown to represent some general speaker-dependent spectral shapes that are effective for modeling speaker identity. The focus is on applications which require high identification rates using short utterance from unconstrained conversational speech and robustness to degradations produced by transmission over a telephone channel. But an increase in telephonic speech utterances causes a decrease in accuracy. Further optimizations are performed on the GMM model by stacking the means of the every GMM model to form a GMM mean supervector [10]. This GMM supervector is used as a parameter in a support vector machine (SVM) classifier.In [11], in order to support speaker verification (SV) in portable devices and in telephone servers with millions of users, Viterbi algorithm is for Hidden Markov model (HMM) alignment is used. Selection of a beam is crucial in however, it is difficult to determine a suitable beam width beforehand. A small beam width may miss the optimal path while a large one may slow down the alignment. A non-heuristic approach is proposed to reduce the search-space. Literature proposes Bayesian approaches used for speaker verification [12]. The verification decision is made by evaluating Bayes factors against a critical threshold. The algorithm aims to apply an efficient algorithm to calculate the Bayes factors for the GMM. But the calculation of the Bayes factors in turn requires the computation of several Bayesian predictive densities. Hence the elliptical basis function parameters were estimated by the EM algorithm with application to speaker verification [13].Speech signals involve phonemes which is a perceptually distinct unit of sound in a specified language that distinguish one word from another. In Whispered Speech, voicing is greatly reduced or eliminated, so the pronunciation of phonemes does not follow the standard pronunciation like that of normal speech, from which the distinctive features were identified. The phonemes are indistinguishable in whispered speech leading to an erroneous word match. Related works on speaker recognition based on whispered speech have addressed the problem in various approaches [14], [15], [16], [17].3. Feature ExtractionThe most usually utilized component extraction technique in speaker acknowledgment is Mel-Frequency Cepstral Coefficients (MFCC). This element extraction technique was first said by Bridle and Brown in 1974 and additionally created by Mermelstein in 1976.MFCC emulates the logarithmic view of tumult and pitch of human sound-related framework and endeavors to wipe out speaker subordinate qualities by barring the crucial recurrence and their music. To speak to the dynamic idea of discourse the MFCC likewise incorporates the difference in the component vector after some time as a major aspect of the element vector. Underneath portrays the procedure for acquiring the MFCC highlight vector.3.1 Pre-emphasisThe initial step is to apply a pre-emphasis filter on the signal to intensify the high frequencies to lessen noise. To avoid numerical problems during the Fourier transform operation, the pre-emphasis filter adjusts the recurrence range since high frequencies have smaller magnitudes compared to lower frequencies.3.2 FramingAfter pre-emphasis, we have to part the signal into short time frames. The rationale behind this progression is that frequencies in a signal, change after some time, subsequently if the Fourier transform is applied over the whole signal, the frequency contours of the signal over time would be lost. To stay away from that, we can securely accept that frequencies in a signal are stationary over a brief time frame. Along these lines, by completing a Fourier change over this brief timeframe outline, we can acquire a decent estimate of the frequency contours of the signal by linking adjoining outlines. We utilize a frame size of 50 milliseconds and a frame step of 25 milliseconds (50% overlap).3.3 WindowingWindowing each individual frame will minimize the spectral distortion to narrow the signal discontinuities for each frame both at the beginning and the end. A Hamming window is provided since it provides a good balance between frequency resolution (the separability of closely grouped peaks in the frequency domain) and dynamic range.3.4 Discrete Fourier Transforms (DFT)To take the Discrete Fourier Transform of the frame, the following is performed:Where is a sample hamming window, and is the length of the Discrete Fourier Transform. The Periodogram based power spectral estimate for the speech frame is given by the Periodogram estimate of the power spectrum:The absolute value of the complex fourier transform is taken and the result is squared. A 512 point DFT is performed and only the first 257 coefficients are kept.3.5 Mel Scaled Filter BanksThe filter banks are figured by applying triangular channels on a Mel-scale to the power range to separate recurrence groups. The Mel-scale intends to mirror the non-straight human ear view of sound by applying little changes in pitch at low frequencies than they are at high frequencies. Joining this scale makes our highlights coordinate all the more intently what people hear.We can convert between Hertz (f) and Mel (m) using the following equations:The formula for converting from frequency to Mel scale is:To go from Mel back to frequency:Each filter in the filter bank is triangular having a response of 1 at the center frequency and decrease linearly towards 0 till it reaches the center frequencies of the two adjacent filters where the response is 0 as shown in figure.A typical MFCC feature vector would be calculated from a window with 512 sample points and consist of 13 cepstral coefficients, 13 first and 13 second order derivatives. Only the first 13(the lower dimensions) of MFCCs are considered since they represent the envelope of spectra and to reduce the dimensionality of the feature space.4. Machine Learning Techniques4.1 Support Vector Machines (SVM)SVM is one of the supervised Machine Learning classification strategies that is broadly connected in the field of speaker recognition. SVM works by selecting samples from all classes known as support vectors, and separating the classes by creating a linear function that partitions them as extensively as conceivable utilizing these support vectors. SVM is principally a classifier technique that performs classsification tasks by developing hyperplanes in a multidimensional space that isolates instances of various class names. Consequently, it goes about as a mapping between an information vector to a high dimensionality space is made utilizing SVM that means to locate the most appropriate hyperplane that partitions the informational collection into classes.[Fig] This linear classifier aims to maximize the distance between the decision hyperplane and the nearest data point, which is called the marginal distance, by finding the best suited hyperplane. SVM depends on the support vectors, which are the data sets closest to the decision boundary, in their algorithms. This is because removing other data points that are further away from the decision hyperplane will not change the boundary as much as if the support vectors were removed.4.2 RANDOM FORESTSRandom Forests [18] is an extension over Bagging(Bootstrap Aggregation) used when the aim is to reduce the variance of a set of decision trees. Subsets of data are created from training sample chosen randomly with replacement. Each collection of subset data is used to train their decision trees. Random selection of features occurs rather than using all features to grow trees. As a result, we end up with an ensemble of different models. Average of all the predictions from different trees are used which is more robust than a single decision tree. The trees are made uncorrelated to maximize the decrease in variance.4.3 K Nearest NeighborsK Nearest Neighbors is a non-parametric classification algorithm. It relegates to an unlabeled sample point, the class of the nearest of a set of previously labeled points named focuses. The rule is independent of the joint distribution of the sample points and their classification. It is appropriate for multi-modal classes and also applications where object can have many labels. The performance is subject to choosing good estimation of k. There is no principled method to choose k, with the exception of through computationally costly systems like cross validation. It is affected by noise and it is sensitive to sensitive features.4.4 EXTRA TreesThe Extra-Trees algorithm builds a group of unpruned choice or regression trees as per the traditional top-down procedure. Its two fundamental contrasts with other tree-based ensemble strategies are that it splits nodes by choosing cut-points completely at random and that it utilizes the entire learning sample (as opposed to a bootstrap replica) to grow the trees. The top-down splitting in the tree is randomized. Rather than computing the locally optimal feature based on information gain or Gini impurity, a random value is selected for the split for each feature under consideration. This value is selected from the features empirical range in the trees training set.4.5 Gradient BoostingBoosting is used to create a collection of predictors. In this technique, learners are learned sequentially with early learners fitting simple models to the data and then analyzing data for errors. Consecutive trees (random sample) are fit and at every step, the goal is to improve the accuracy from the prior tree. When an input is misclassified by a hypothesis, its weight is increased so that next hypothesis is more likely to classify it correctly. This process converts weak learners into better performing model. Upon combining gradient descent and boosting, gradient boosting is formed. At each iteration, a regression tree model is fitted to predict the negative gradient by allowing optimization of an arbitrary differentiable loss function.5. Proposed Speaker recognition systemSpeaker recognition systems consists of two main modules: feature extraction and feature matching. Feature extraction is the process of extracting a small amount of data from the voice signal that is used to represent each speaker. The feature vector signifies specific characteristics of the speaker. There exists various feature extraction techniques like Linear Prediction Coding (LPC), Mel Frequency Cepstral Coefficients (MFCC), Perceptual Linear Predictive Coefficients (PLP) and many more that are used. Analysis of the feature extraction methods [19] led to the selection of MFCC (Mel Frequency Cepstral Coefficients) as the feature extraction technique to be utilized, to facilitate better feature extraction by reducing the noise [20]. Feature matching involves the process of identifying the unknown speaker by comparing the extracted features from his/her voice input with the ones from a set of known speakers. Feature Matching is implemented through the machine learning algorithms.6. Performance measures and Experimental results6.1 Performance measures:For the evaluation metric, Accuracy, Precision, Recall and F-Measure are utilized as performance metrics. Accuracy corresponds to the proportion of speakers that are correctly recognized. Precision indicates the proportion of positive identifications that are actually correct. Recall indicates the proportion of actual positives that are identified correctly. F- Measure is the harmonic mean of precision and recall. It could be viewed as a summary measure that combines both precision and recall. F-Measure evaluates, if an increase in precision (recall) outweighs a reduction in recall (precision). The table is the confusion matrix.The precision, recall and F-Measure are computed using the formula [Fig].The fig. Describes the metrics computed6.2 Experimental results and Discussion:6.2.1 DatasetThe CHAracterizing INdividual Speakers (CHAINS) [21] Whispered Speech Corpus consists of 36 speakers, is utilized for the process of Speaker Recognition. It consists of 28 speakers (14 male, 14 female) of those are from the Eastern part of Ireland, and speak Eastern Hiberno-English. The remaining 8 speakers (4 male, 4 female) are from the UK and the USA. A whispered speech signal is taken as an input and its feature vectors are extracted which characterizes the particular signal. These feature vectors are unique for each speaker. These are used to identify the speaker during the testing phase by matching the features of a known speaker with the unknown sounds.6.2.2 Experiment SetupStratified 10-fold cross validation is used to evaluate the learning algorithms. For the stratified 10-fold cross validation, we randomly divide the dataset into 10 folds, and 9 folds are used to train the classifier, while the remaining 1 fold is used to evaluate the performance, and the class distribution in training and test set is the same as the original dataset to simulate the actual usage of the algorithm. The execution of the feature extraction and feature matching utilize the pyAudioAnalysis [22] library.The implementation occurs through the learning algorithms: Gradient Boosting, EXTRA Trees, Random Forests, Support Vector Machines and K-Nearest Neighbors. Every algorithm uses parameters designatedThe soft margin parameter C for the SVM classifierThe number of nearest neighbors, k for the kNN classifier.The number of trees in the random forest classifier.The number of boosting stages in the gradient boosting classifier.The number of trees in the forest of the extra trees classifier.6.2.1 Support Vector Machine Performance:In a SVM, two variations exist for selecting a hyperplane: a hyperplane with the largest minimum margin, and a hyperplane that correctly separates as many instances as possible. The problem is that both the objectives cannot be achieved completely. The C parameter determines how much the latter objective is necessary that is how much one wants to avoid misclassifying each training example. For large values of C, the optimization will choose a smaller-margin hyper-plane, if the selected hyper-plane is capable of getting all the training points classified correctly. Conversely, a very small value of C will cause the optimizer to look for a larger-margin separating hyper-plane, even if that hyper-plane misclassifies more points. For very small values of C, misclassified examples are often obtained, even if the training data is linearly separable. The choice of C value depends on what the future data looks like.For initial small values (0.001), presence of highly misclassified samples leads to less accuracy. A gradual increase in C value still keeping the value low, an increase in accuracy is noted.SOFT MARGIN CACCURACYF- MEASURE0.0016766.80.0185.385.20.585.785.81.086865.085.585.610.085.885.920.086.686.6The data we observe is the depiction of using a low C which gives a low minimum margin. The outliers are neglected and thus end up with a much smaller margin. On the right there is a high C used. There is an increase in outliers indicating an increase in the number of elements classified incorrectly. Thus 20.0 as the C value provides the highest accuracy and F-measure.6.2.2 Random Forest PerformanceIn general, ensemble methods reduces the prediction variance to almost nothing, improving the accuracy of the ensemble. The variance of the expected generalization error of an individual randomized model is defined as:The variance of the expected generalization error of an ensemble for predictor is given by as:Where p(x) is the Pearsons correlation coefficient between the predictions of two randomized models trained on the same data from two independent seeds. An increase in the number of decision trees in the random forest implying a larger M causes the variance of the ensemble to decrease, when ?(x) is less than 1. Therefore, the variance of an ensemble is strictly smaller than the variance of an individual model. Increasing the number of individual randomized models in an ensemble does not increase the generalization error. Thus, an increase in the number of trees causes a decrease in variance. Small variance and little bias thus lead to a highly accurate estimator. Hence parameter 500 indicates the number of trees among the parameters for the highest accuracy.PARAMETERACCURACYF-MEASURE10.00060.36625.00070.069.450.00074.574.0100.00077.376.8200.00079.278.6500.00080.980.46.2.3 EXTRA Trees PerformancePARAMETERACCURACYF-MEASURE10.00056.455.825.00069.568.950.00075.274.7100.00078.277.8200.00080.579.9500.00081.380.8The parameter indicates the number of decision trees in the forest. The main objective of further randomizing tree building in the context of numerical input features is, where the choice of the optimal cut-point is responsible for a large proportion of the variance of the induced tree. From a statistical point of view, dropping the bootstrapping idea leads to an advantage in terms of bias, whereas the cut-point randomization has often an excellent variance reduction effect. An increase in the number of trees indicates a decrease in variance. Low variance infers high precision leading to a higher accuracies. Thus an increase in the number of trees leads to increased accuracy.6.2.4 K- NEAREST NEIGHBOR PerformanceThe parameter indicates the number of returned nearest neighbors. The K returned neighbors have an impact on the accuracy. There is normally a K value that gives good performance depending on application which is determined by the receiver operating characteristic (ROC) curve, to find a suitable K value. Normally K = 1 is too noisy, and to smooth things out K = 3 or 5 are used. However, larger K values will tend to bias the KNN algorithm towards a dominant class, thus causing a situation of overfitting. As observed, an increase in K value to a particular limit causes increase in accuracy. But for larger k values, an increase in bias leads to decreased accuracy. Hence for k=5.000, the highest accuracy is obtained.PARAMETERACCURACYF-MEASURE1.00070.070.23.00069.469.65.00071.971.87.00070.870.69.00071.471.111.00070.870.413.00069.769.215.00071.070.56.2.5 Gradient Boosting PerformanceThe most important two are the number of trees and the learning rate. The parameter indicates the number of the trees implemented. The learning rate is fixed at 0.1. . For a particular learning rate, to find the optimum number of trees. As observed, with the increase in the number of trees, the accuracy increases to obtain highest accuracy at number of trees set to 500 among the parameters.PARAMETERACCURACYF-MEASURE10.00056.056.425.00060.360.950.00066.066.5100.00067.668.0200.00069.169.3500.00069.569.5Conclusion and Future WorkThis paper discusses the most commonly used supervised machine learning algorithms for classification. Our aim was to prepare a comprehensive review of the key ideas, drawing out pros and cons and useful variants of the discussed algorithms. The paper shows that every algorithm differs according to area of application and it is not the case that a single algorithm is superior in every scenario. The decision of choosing an appropriate algorithm is based on the type of problem and the data available. Again, by choosing two or more suitable algorithm and creating an ensemble, the accuracy can be increased. We hope that the references cited cover the major drawbacks, guiding the researcher in interesting research directionsREFERENCES1. K. Jain, A. Ross, and S. Prabhakar, An introduction to biometric recognition, IEEE Trans. Circuits Syst. Video Technol., vol. 14, no. 1, pp. 420, Jan. 20042. L. Rabiner, B.H. Juang, Fundamentals of Speech Recognition, Prentice Hall, 1993.[3] Wan, V., Campbell, W.M., 2000. Support vector machines for verification and identification, in: Neural Networks for Signal Processing X, Proceedings of the 2000 IEEE Signal Processing Workshop, pp. 775784.[4] Ganapathiraju, A., Picone, J., 2000. Hybrid SVM/HMM architectures for speech recognition, in: Speech Transcription Workshop[5]John C. Platt,1999. Probabilistic Outputs for Support Vector Machines and Comparisons to Regularized Likelihood Methods, ADVANCES IN LARGE MARGIN CLASSIFIERS, MIT Press.[6] J. C. Wang, C. H. Yang, J. F. Wang, and H. P. Lee, Robust speaker identification and verification, IEEE Comput. Intell. Mag., vol. 2, no. 2, pp. 5259, May 2007.[7] W. M. Campbell, J. P. Campbell, D. A. Reynolds, E. Singer, and P. A. Torres-Carrasquillo, Support vector machines for speaker and language recognition, Comput. Speech Language, vol. 20, no. 23, pp. 210229, Apr.-Jul. 2006. [8] D. A. Reynolds and R. C. Rose, Robust text-independent speaker identification using Gaussian mixture speaker models, IEEE Trans. Speech Audio Process., vol. 3, no. 1, pp. 7283, Jan. 1995.[9] Xiang and T. Berger, Efficient text-independent speaker verification with structural Gaussian mixture models and neural network, IEEE Trans. Speech Audio Process., vol. 11, no. 5, pp. 447456, Sep. 2003.[10] W. M. Campbell, D. E. Sturim, and D. A. Reynolds, Support vector machines using GMM supervectors for speaker verification, IEEE Signal Process. Lett., vol. 13, no. 5, pp. 308311, May 2006.[11] Q. Li, A detection approach to search-space reduction for HMM state alignment in speaker verification, IEEE Trans. Speech Audio Process., vol. 9, no. 5, pp. 569578, Jul. 2001.[12] H. Jiang and L. Deng, A Bayesian approach to the verification problem: Applications to speaker verification, IEEE Trans. Speech Audio Process., vol. 9, no. 8, pp. 874884, Nov. 2001.[13] M. W. Mak and S. Y. Kung, Estimation of elliptical basis function parameters by the EM algorithm with application to speaker verification, IEEE Trans. Neural Netw., vol. 11, no. 4, pp. 961969, Jul. 2000. [14] Q. Jin, S. S. Jou, and T. Schultz, Whispering speaker identification, in Proc. IEEE Int. Conf. Multimedia Expo, 2007, pp. 10271030.[15] J. Gu and H. M. Zhao, Whispered speech speaker identification based on SVM and FA, in Proc. Int. Conf. Audio Language Image Processing, Nov. 2325, 2010.[16] J. Xu and H. Zhao, Speaker identification with whispered speech using unvoiced-consonant phonemes, in Proc. Int. Conf. Image Anal. Signal Process, Nov. 911, 2012.[17] Jia-Ching Wang, Senior Member, IEEE, Yu-Hao Chin, Wen-Chi Hsieh, Chang-Hong Lin, Ying-Ren Chen, and Ernestasia Siahaan, Speaker Identification With Whispered Speech for the Access Control System in IEEE TRANSACTIONS ON AUTOMATION SCIENCE AND ENGINEERING, VOL. 12, NO. 4, OCTOBER 2015.[18] Leo Breiman. Random forests. Machine Learning Journal, 45:532, 2001.[19] Shahzadi Farah, Azra Shamim,Speaker recognition system using mel-frequency cepstrum coefficients, linear prediction coding and vector quantization, 2013 3rd IEEE International Conference on Computer, Control and Communication (IC4).[20]Syed Sibtain Khalid, Safdar Tanweer, Dr. Abdul Mobin, Dr.Afshar Alam, A comparative Performance Analysis of LPC and MFCC for Noise Estimation in Speech Recognition Task, International Journal of Electronics Engineering Research, Volume 9, Number 3, 2003.[21]Cummins, Fred, et al. CHAracterizing INdividual Speakers (CHAINS) LDC2008S09. Web Download. Philadelphia: Linguistic Data Consortium, 2008.[22]Giannakopoulos T (2015) pyAudioAnalysis: An Open-Source Python Library for Audio Signal Analysis. PLoS ONE 10(12): e0144610.
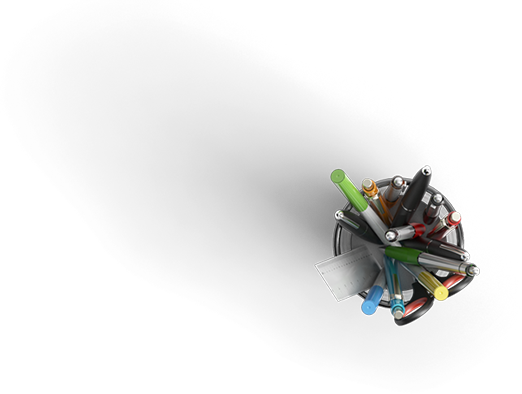



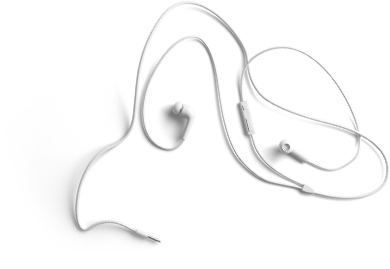
Comparative Analysis of Machine Learning Algorithms in Speaker Recognition of Whispered Speech
Mar 17, 2020 | Sciences
Our Service Charter
1. Professional & Expert Writers: Topnotch Essay only hires the best. Our writers are specially selected and recruited, after which they undergo further training to perfect their skills for specialization purposes. Moreover, our writers are holders of masters and Ph.D. degrees. They have impressive academic records, besides being native English speakers.
2. Top Quality Papers: Our customers are always guaranteed of papers that exceed their expectations. All our writers have +5 years of experience. This implies that all papers are written by individuals who are experts in their fields. In addition, the quality team reviews all the papers before sending them to the customers.
3. Plagiarism-Free Papers: All papers provided by Topnotch Essay are written from scratch. Appropriate referencing and citation of key information are followed. Plagiarism checkers are used by the Quality assurance team and our editors just to double-check that there are no instances of plagiarism.
4. Timely Delivery: Time wasted is equivalent to a failed dedication and commitment. Topnotch Essay is known for timely delivery of any pending customer orders. Customers are well informed of the progress of their papers to ensure they keep track of what the writer is providing before the final draft is sent for grading.
5. Affordable Prices: Our prices are fairly structured to fit in all groups. Any customer willing to place their assignments with us can do so at very affordable prices. In addition, our customers enjoy regular discounts and bonuses.
6. 24/7 Customer Support: At Topnotch Essay, we have put in place a team of experts who answer to all customer inquiries promptly. The best part is the ever-availability of the team. Customers can make inquiries anytime.
Recent Posts
- Positive Psy-5.1 Discerning Truth Getting Started
- Given your understanding of the functions of the different lobes of the brain, how might a person be affected by damage to the temporal lobe?
- History Discussion Assignment
- Discussion: Explore how lifestyle changes-such as nutrition–can prevent or modify disease processes
- Read the article on Spirit Airlines
Archives
- January 2025
- August 2024
- July 2024
- June 2024
- May 2024
- April 2024
- January 2024
- December 2023
- August 2023
- July 2023
- June 2023
- May 2023
- January 2023
- October 2022
- September 2022
- August 2022
- July 2022
- June 2022
- May 2022
- March 2022
- February 2022
- January 2022
- December 2021
- November 2021
- October 2021
- September 2021
- August 2021
- July 2021
- June 2021
- May 2021
- April 2021
- March 2021
- February 2021
- January 2021
- December 2020
- November 2020
- October 2020
- September 2020
- August 2020
- July 2020
- June 2020
- May 2020
- April 2020
- March 2020
- February 2020
- January 2020
- December 2019
- November 2019
- October 2019
- September 2019
Categories
- `
- .uul l illl'
- "" ""Qualitative Analysis Assignment: Transcript 3
- "" which is attached to this assignment.
- (15 points) Lot Ai? ?Xn be a random sample from a f/(0,0) population with unknown parameter 0 > 0. Find the likelihood ratio test (LRT) of size a for testing Ho : 0 > 0q .vs. H : 0 < Oq.
- { ""cell_type"": ""markdown""
- /files/-np-ex19-7b-xlsx
- /files/0886109913504154-pdf,/files/1363460706065057-pdf,/files/0886109914560741-pdf
- /files/11357-txt,/files/cavell-pdf
- /files/20191118150623part-vii-assessment-tool-development-rub1-pdf
- /files/20191119051422iom-report-future-of-nursing-rubric-xlsx
- /files/20200428035024defend-or-refute-guide-pdf
- /files/adjustmentdisorder-pdf,/files/depression1-pdf-6973733
- /files/assignment1j-docx-7815535,/files/assignment2j-docx
- /files/assignment2-docx-5634861,/files/networking14-pkt,/files/20200204-routers-table-docx,/files/20200204-network-infrastructure-design-pdf-5640375
- /files/assignment2-docx-8830717
- /files/bookaccess-docx-5088805
- /files/bookchap1-412-pdf,/files/bookchap1-412-pdf-7977187
- /files/businessculturaldimensionsanalysisgradingrubric-pdf-7789673"
- /files/casestudy2-docx-5603093
- /files/chapter5-pdf-7822037
- /files/crjs3001wk1studentreplies-docx
- /files/developingobjectives1-docx,/files/article3assignments3-41-pdf,/files/developingobjectives1-docx-7919359
- /files/discussionreflectionguide-docx,/files/introduction-to-criminology-10th-edition-9781544339023-pdf,/files/sample-docx-7598097
- /files/essay2-documentedargumentrevised22-pdf-6947741
- /files/frenchandindianwar-docx-7926377,/files/bostonteapartyaftermath-docx
- /files/homework2negotiationmorgan-docx,/files/homeworknegotiation1michele-docx
- /files/labassignmnetw8-docx-7665133
- /files/lessonweek4-docx
- /files/mat240houselistingpricebyregionpjct5-xlsx-7794861,/files/mat240modulefiveassignmenttemplatepjct5-docx-7794863
- /files/microm53question-docx
- /files/milecture-pdf,/files/motivationalinterviewing-thebmj-pdf
- /files/nrnpprac6635comprehensivepsychiatricevaluationtemplate-docx-8263023"
- /files/principlesofcorporatefinance13erichardbrealey-pdf-7629311,/files/wk71-docx,/files/wk72-docx,/files/wk73-docx,/files/wk74-docx
- /files/problemstatementchecklist-pdf-6967979,/files/week4article-pdf-6967981
- /files/prospectusfinaldraft-correctedversion-docx,/files/dissertationprospectusoriginalversion-docx
- /files/reflectionpaperguidelines-docx-7570139
- /files/rubricpandv-pdf-7584061,/files/page11-pdf-7584063,/files/page9-10-pdf-7584065,/files/implicationofpiagetsandvygotskytheories-summer2021socialstudiesmethodsforece-fieldece-3571-01-pdf
- /files/screenshot2021-02-27at11-35-38am-png"
- /files/sec-516-t6-meetingtheneedsofdiverselearners-docx"
- /files/spd-400-d-t6-studentgoalsandinterventionplantemplate-docx-7938583,/files/rub-docx-7938623
- /files/taskdifficultyandincubation1-docx
- /files/unitvinternationlstudyguide-pdf-7796117
- /files/visualcommunicationassessment23051-docx
- /files/week3assignmentg-docx
- /files/week4rubric-docx-7856191
- /files/week7readingdestructivebehavior-pdf-7890761,/files/bibliu-print-9781452236315table143-pdf
- /files/westernphilosophyananthologybycottinghamjohnz-lib-org-pdf"
- /files/woodch9-pdf,/files/chapter8-pdf-6111751
- /files/youthexposedtoviolenceindc-august2021-docx,/files/chapteronethenatureandtoolsofresearch-pdf
- ~I'vcrn I""theorems"" follow from it
- 1111 writing and reading is embedded in some Discourse
- 12 font
- 12 point font
- 2015
- 2021
- 2021). HTML pages are an excellent example that allows network shares hence makes the embedding of the resources possible. Therefore
- 3 PAGE ESSAY ON WHY I DONT WANT ANY TATTOOS. I NEED CLEAR EXAMPLES
- 4-6sentence · Sentence1-2:Author + Genre + Context of the Bible book (that the story is a part of) · Sentences 3-5: Brief Summary Including Story Genre (historical narrative, mythology, or parable).
- 4)."
- Acc 206
- ACC 206, BUSINESS FINANCE
- ACC201
- ACC544, BUSINESS FINANCE
- ACC556
- ACC573, Business & Finance
- ACC60171, Other
- Accounting & Finance
- Acct 101
- ACCTG406
- ACIS5104
- ACOL202
- acquire (at least) one initial Discourse. This initial Discourse
- actively engaging with course materials
- acts
- AD712, Business & Finance
- adding beliefs
- ADMIN565
- ADMN575, OTHER
- allocating specific time slots for coursework
- ALY6100
- American Military University
- AMERICAN MILITARY UNIVERSITY, BUSINESS FINANCE
- AMERICAN MILITARY UNIVERSITY, WRITING
- American Public University System
- AMERICAN PUBLIC UNIVERSITY SYSTEM, BUSINESS FINANCE
- AMERICAN PUBLIC UNIVERSITY SYSTEM, HUMANITIES
- AMH2020
- analyze and evaluate the following questions:
- and cost were controlled.
- and academic grammar and usage.
- and architecture the guys work execution at the program or system level. At the risk of falling victim to stating the obvious
- and describe the type of economic analysis that you would use in the evaluation.
- and external resources recommended by instructors. Utilize online libraries
- and interactive components. Take comprehensive notes
- and managing your time effectively
- and often write
- and personal health record (PHR)
- and related terms for two separate concepts. (examples: technology-computer
- and Transportation and Telecommunication. Using The World Factbook
- and users are able to inject SQL commands using the available input (Imperva
- ANM104
- ANM104 OL1
- ANTH130, SCIENCE
- ANTHROP 2200
- Anthropology 130
- Applied Science
- Applied Sciences
- Applied SciencesApplied Sciences
- Architecture and Design
- Architecture and DesignArchitecture and Design
- Arizona State University
- ARIZONA STATE UNIVERSITY, WRITING
- Art
- ART101
- ART2010
- ARTH102
- article
- Arts
- ARTS1301
- ASC400, BUSINESS FINANCE
- ashford university
- ASHFORD UNIVERSITY, BUSINESS FINANCE
- ASHFORD UNIVERSITY, HUMANITIES
- ASHFORD UNIVERSITY, OTHER
- ASHFORD UNIVERSITY, SCIENCE
- Ashworth College
- asking thoughtful questions and providing constructive feedback to your peers. Regularly check your course emails and notifications
- assignments
- at least
- Atlantic International University Online, Science
- attitudes
- BADM735
- BAM515, BUSINESS FINANCE
- BCJ3601, BUSINESS FINANCE
- BCN4431
- BEHS380, WRITING
- Berkshire Community College
- Bethel University
- BETHEL UNIVERSITY, HUMANITIES
- beyond the family and immediate kin and peer group. These may be 1111.
- BHR3352
- BHR3352 Human Resource Management
- BIO1100
- BIO1408
- BIO2401
- BIO3320
- BIO354, SCIENCE
- BIOCHEM202
- Biology
- Biology – Anatomy
- Biology – AnatomyBiology – Anatomy
- Biology – Ecology
- Biology – Physiology
- BIOLOGY 10, SCIENCE
- BiologyBiology
- Blog
- BME351
- body
- Bowie State University
- Bowie State University, Science
- brings with it the (poten- u.il) acquisition of social ""goods"" (money
- BROCK UNIVERSITY, BUSINESS FINANCE
- BROCK UNIVERSITY, OTHER
- BROOKDALE COMMUNITY COLLEGE, HUMANITIES
- BROOKLYN COLLEGE, WRITING
- BUAD326, Business & Finance
- BULE303
- BUS1001
- BUS120, Business & Finance
- BUS125, WRITING
- BUS187, Business & Finance
- BUS232
- BUS242
- BUS303, BUSINESS FINANCE
- BUS410, BUSINESS FINANCE
- BUS472, SCIENCE
- BUS475
- BUS499
- BUS520, Business & Finance
- BUS530
- BUS542
- BUS599
- BUS620
- BUS623
- BUS630
- BUSI320
- Business
- Business – Management
- Business & Finance
- Business & Finance – Financial markets
- Business & Finance – Financial marketsBusiness & Finance – Financial markets
- Business & Finance – Marketing
- Business & Finance – MarketingBusiness & Finance – Marketing
- Business & Finance – Supply Chain Management
- Business & Finance , BUS430
- Business & Finance , BUSN370
- Business & Finance , COMM240
- Business & Finance , COMS2302
- Business & Finance , ENT527
- Business & Finance , FIRE3301
- Business & Finance , G141COM1002
- Business & Finance , GB520
- Business & Finance , GB540
- Business & Finance , IBSU487
- Business & Finance , JWI515 Managerial Economics
- Business & Finance , MGT16
- Business & Finance , MGT496
- Business & Finance , MGT498
- Business & Finance , MGT521
- Business & Finance , MT460
- Business & Finance , PM586
- Business & Finance , RMI3348
- Business & Finance , SOC450
- Business & Finance , south university online
- Business & Finance , Strayer University
- Business & Finance , University of Phoenix
- Business & Finance , Wilmington University
- Business & Finance, Trident University
- Business & FinanceBusiness & Finance
- Business and Finance
- Business Finance – Accounting
- Business Finance – AccountingBusiness Finance – Accounting
- Business Finance – Economics
- Business Finance – EconomicsBusiness Finance – Economics
- Business Finance – Management
- Business Finance – ManagementBusiness Finance – Management
- Business Finance – Operations Management
- Business Finance – Operations ManagementBusiness Finance – Operations Management
- BUSINESS FINANCE, CBBU1001
- BUSINESS FINANCE, COLORADO STATE UNIVERSITY GLOBAL
- BUSINESS FINANCE, COLORADO TECHNICAL UNIVERSITY
- BUSINESS FINANCE, COLUMBIA SOUTHERN UNIVERSITY
- BUSINESS FINANCE, COM 510
- BUSINESS FINANCE, CRJ101
- BUSINESS FINANCE, DOC660
- BUSINESS FINANCE, EASTERN KENTUCKY UNIVERSITY
- BUSINESS FINANCE, ECN 501
- BUSINESS FINANCE, ECO2251
- BUSINESS FINANCE, ECO531
- BUSINESS FINANCE, FIN 500
- BUSINESS FINANCE, FIN31FMS12019
- BUSINESS FINANCE, GRANTHAM UNIVERSITY
- BUSINESS FINANCE, HLS3302
- BUSINESS FINANCE, HRC164
- BUSINESS FINANCE, HRM 500
- BUSINESS FINANCE, INDS 400
- BUSINESS FINANCE, INT113
- BUSINESS FINANCE, INTL3306
- BUSINESS FINANCE, ISDS 351
- BUSINESS FINANCE, LAWS OF EVIDENCE
- BUSINESS FINANCE, LIBERTY UNIVERSITY
- BUSINESS FINANCE, MAN3504
- BUSINESS FINANCE, MBA 5121
- BUSINESS FINANCE, MG260
- BUSINESS FINANCE, MGMT386
- BUSINESS FINANCE, MGT 521
- BUSINESS FINANCE, MGT211
- BUSINESS FINANCE, MICHIGAN STATE UNIVERSITY
- BUSINESS FINANCE, MIDDLE TENNESSEE STATE UNIVERSITY
- BUSINESS FINANCE, MKT331
- BUSINESS FINANCE, MKT419
- BUSINESS FINANCE, NORTHEAST MONTESSORI INSTITUTE
- BUSINESS FINANCE, OAKLAND UNIVERSITY
- BUSINESS FINANCE, PARK UNIVERSITY
- BUSINESS FINANCE, RASMUSSEN COLLEGE
- BUSINESS FINANCE, SOUTHERN NEW HAMPSHIRE UNIVERSITY
- BUSINESS FINANCE, TRIDENT INTERNATIONAL UNIVERSITY
- Business Strategy
- C11E
- Calculus
- California Baptist University
- California Coast University
- CALIFORNIA STATE UNIVERSITY NORTHRIDGE, OTHER
- can be studied
- can never 21 really be liberating literacies. For a literacy to be liberating it must contain both the Discourse it is going to critique and a set of meta-elements (language
- Capella University
- Capella University, Humanities
- Capital L. George Adams
- CATEGORY
- CE304
- CE445
- CEE792
- CEGR338
- Chamberlain College of Nursing
- Chapter 3
- Charles R Drew University of Medicine and Science
- charles sturt university
- Chaudhary Charan Singh University, Humanities
- CHEM101
- CHEM111
- CHEM1411, Science
- CHEM202, Science
- CHEM210, Science
- CHEM410
- Chemistry
- Chemistry – Chemical Engineering
- Chemistry – Organic chemistry
- Chemistry – Pharmacology
- Chemistry – Physical chemistry
- ChemistryChemistry
- Childcare
- CHMY373, SCIENCE
- Choose three problematic issues that are currently facing older people living in the community?
- CINE286U
- CIS210
- cis273
- CIS359
- CIS510, Other
- CIS524
- CIVL6603, Science
- CJ430, SCIENCE
- CJA444
- CJUS300, Other
- Classics
- CMIT495
- CMSC140
- Colorado Christian University
- COLORADO STATE UNIVERSITY GLOBAL, SCIENCE
- COLORADO STATE UNIVERSITY, SCIENCE
- Colorado State UniversityGlobal
- Colorado Technical University
- COLORADO TECHNICAL UNIVERSITY, OTHER
- Colorado Technical University, Programming
- Columbia Southern University
- COLUMBIA SOUTHERN UNIVERSITY, OTHER
- Columbia Southern University, Science
- COLUMBIA SOUTHERN UNIVERSITY, WRITING
- Commerce
- Communication
- Communications
- COMMUNITY-BASED CORRECTIONS
- COMP1007
- Computer Science
- Computer Science – Java
- Computer Science- Python
- Computer ScienceComputer Science
- Construction
- correctness
- Cosc1437
- counseling chemical dependency adolescents
- Criminology
- CRJ305
- cross-site scripting
- CS101
- CSIT 100, PROGRAMMING
- CSPM326
- CST 610, PROGRAMMING
- Cultural Studies
- culturally appropriate intervention to address childhood obesity in a low-income African American community.
- CUR535
- CUYAMACA COLLEGE, HUMANITIES
- CUYMACA COLLAGE, HUMANITIES
- Data Analysis
- DAVIDSON COLLEGE, OTHER
- defensive programming allows for more efficient processes while also protecting systems from attack.
- DES201
- Describe the difference between glycogenesis and glycogenin ? Explain in 10 to 12 lines.
- Design
- Digital Marketing Plan for Nissan Motor Co. The plan will identify the current marketing opportunity and/or problem(s) and propose digital marketing solutions. Please use header in the attachment."
- Discuss one way in which the Soviet Union fulfilled communist thought, and another way in which it did not with reference to O'Neil's Chapter 9.
- Discuss the pros and cons of free-market based economies and how they impact the modern, globalized economy? What comes to your mind when you hear the term "globalization?"
- Dissertation
- DMM612, Science
- DMM649, SCIENCE
- Draft and essay of 1,000 words minimum, stating the Most Important and Relevant aspects to be considered when carrying on INTERNATIONAL NEGOTIATIONS or MULTI-CULTURAL NEGOTIATIONS.
- Drama
- each time a user extracts the ZIP file
- Earth Science – Geography
- Earth Science – GeographyEarth Science – Geography
- Earth Science – Geology
- EAS1601
- ECD 541, HUMANITIES
- ECE 452
- Ecommerce
- ECON335
- Economics
- ECPI University
- EDUC696
- Education
- EducationEducation
- EEL3472C
- EEL3705
- EET110
- EFFAT UNIVERSITY JEDDAH, HUMANITIES
- elasticity
- ELI2055A
- EMDG 230, SCIENCE
- Emglish
- Emory University
- Employment
- EN106
- EN106, HUMANITIES
- EN109
- EN206, HUMANITIES
- ENC1102, Writing
- eng 100
- ENG100
- ENG101
- ENG101, Humanities
- ENG102
- ENG102, Humanities
- eng106
- ENG1102, WRITING
- ENG124
- ENG124, Humanities
- ENG124, Writing
- ENG1340, HUMANITIES
- ENG200, Humanities
- ENG207
- eng2206
- ENG2211
- ENG305
- ENG812
- Engineering
- Engineering – Chemical Engineering
- Engineering – Civil Engineering
- Engineering – Civil EngineeringEngineering – Civil Engineering
- Engineering – Electrical Engineering
- Engineering – Electrical EngineeringEngineering – Electrical Engineering
- Engineering – Electronic Engineering
- Engineering – Mechanical Engineering
- Engineering – Mechanical EngineeringEngineering – Mechanical Engineering
- Engineering – Telecommunications Engineering
- EngineeringEngineering
- ENGL 120
- ENGL 124, OTHER
- ENGL 124, WRITING
- ENGL 2030, HUMANITIES
- ENGL1102
- ENGL120, HUMANITIES
- ENGL120SP2019, WRITING
- ENGL126
- ENGL1302
- ENGL130E, HUMANITIES
- ENGL147N, HUMANITIES
- ENGL2, Humanities
- English
- English – Article writing
- English – Article writingEnglish – Article writing
- English Language
- English Literature
- EnglishEnglish
- ENGR350
- ENST202CORE274
- ensuring you allocate dedicated time for coursework
- Environment
- Environmental Science
- Environmental Sciences
- Environmental Studies
- especially for a small company
- Essay Writing
- etc¦). Please note at least five organizational activities and be specific when responding.
- ETH321
- ETHC445N
- Ethnic Studies 101
- European Studies
- EXNS6223
- Family
- Fashion
- February 20). What is defensive programming? EasyTechJunkie. Retrieved December 30
- film industry
- FILM INDUSTRY, HUMANITIES
- Film Studies
- FIN 500
- FIN330, MATHEMATICS
- FIN370
- Final Essay
- Find the uniform most powerful level of alpha test and determine sample size with the central limit theorem
- Florida International University
- Florida National University
- Florida State College at Jacksonville
- FoothillDe Anza Community College District
- Foreign Languages
- Foreign Languages – Spanish
- formulations
- from https://www.pcmag.com/news/fat32-vs-ntfs-choose-your-own-format
- G124/enc1101
- Gallaudet University
- General Studies
- General_Business
- GEO1206
- GEOG100, Science
- Geography
- GEOL3200, HUMANITIES
- Geometry
- George Mason University
- GERM1027
- GERO 101, SCIENCE
- GERON101
- GLG101, Science
- GO16
- Government
- GovernmentGovernment
- GOVT2305
- GOVT2305, Humanities
- GOVT2306
- Grand Canyon University, Science
- Grand Canyon University, Writing
- Grantham University
- GRANTHAM UNIVERSITY, PROGRAMMING
- GRANTHAM UNIVERSITY, WRITING
- GROSSMONT COLLEGE, HUMANITIES
- Grossmont-Cuyamaca Community College District
- GROSSMONT-CUYAMACA COMMUNITY COLLEGE DISTRICT, HUMANITIES
- GU299, WRITING
- Hawaii Pacific University
- HC310
- HCA415
- HCA521
- HCM550, SCIENCE
- Hcs370
- HCS446
- he focused on aspects of the U.S. that combined democratic and increasingly capitalist characteristics. THINK ABOUT the points De Tocqueville made.
- Health & Medical
- Healthcare
- HIM 2588, MATHEMATICS
- HIM 500, SCIENCE
- HIM301
- HIS 108
- HIS101
- HIS105
- HIS200
- HIST104A, Humanities
- HIST111
- HIST1301, HUMANITIES
- HIST1302
- HIST1320
- HIST1700
- HIST2620
- HIST350, Humanities
- HIST405N, HUMANITIES
- HIST459, Humanities
- History
- History – American history
- History – American historyHistory – American history
- History – Ancient history
- History – Ancient historyHistory – Ancient history
- History – World history
- History – World historyHistory – World history
- HISTORY4250, Humanities
- HistoryHistory
- HLSS508, OTHER
- HMP403
- Hospitality
- HOST1066, WRITING
- Housing
- How do the changes in ship technology effect port operations? Discuss at least 3 factors contributing to port operations and development. Address cargo and passenger liners.250 words
- How have Mary Calderone, SIECUS and other sex educators changed how sex education is perceived? (100 words minimum)
- HOWARD UNIVERSITY, SCIENCE
- HR Management
- HRM300
- HRT6050, Writing
- HSA305
- HSA535
- HSC3201
- HSN476
- HUM1002
- HUM115
- HUM115, Writing
- Human Resource
- Human Resource Management
- Human Resource ManagementHuman Resource Management
- Human Resources
- HUMAN RESOURCES DEVELOPMENT AND MANAGEMENT, SCIENCE
- Human Rights
- HUMANITIES
- Humanities, Alcorn State University
- HUMANITIES, HY 1110
- Humanities, LMC3225D
- HUMANITIES, LONG BEACH CITY COLLEGE
- HUMANITIES, MUSIC1306
- HUMANITIES, OAKLAND COMMUNITY COLLEGE
- HUMANITIES, PH 100
- HUMANITIES, POINT LOMA NAZARENE UNIVERSITY
- HUMANITIES, PRINCE GEORGE'S COMMUNITY COLLEGE
- Humanities, PSY105
- HUMANITIES, PSY330 THEORIES OF PERSONALITY
- Humanities, PSYC 1101
- HUMANITIES, PSYCH305
- HUMANITIES, PSYCH635 PSYCHOLOGY OF LEARNING
- HUMANITIES, RSCH8110
- HUMANITIES, SAN DIEGO STATE UNIVERSITY
- HUMANITIES, SAN JACINTO COLLEGE
- Humanities, SOC1010
- HUMANITIES, SOC401
- HUMANITIES, SOCIOLOGY OF RELIGION
- HUMANITIES, SOUTHERN NEW HAMPSHIRE UNIVERSITY
- HUMANITIES, STRAYER UNIVERSITY
- HUMANITIES, SWK110
- HUMANITIES, UNIVERSITY OF CALIFORNIA
- HUMANITIES, UNIVERSITY OF CALIFORNIA IRVINE
- HUMANITIES, UNIVERSITY OF HOUSTON-DOWNTOWN
- Humanities, University of Maryland University College
- i need the attached work to look like this. please redo and make it look like this.
- I need these questions answered fully. I have the assignment and the notes attached for it. Do not use chegg or course hero. This is due Wednesday 4/14 at 10:00 pm which is almost 4 full days. Thanks!
- I need to re organize a research paper I attached all my information and I attached you an example how is going to be. Please follow the instruction and the references has to be APA 7edition
- Identify a cardiac or respiratory issue and outline the key steps necessary to include for prevention and health promotion
- identify the leadership theory that best aligns with your personal leadership style
- if you suggest trying to do this
- IGLOBAL UNIVERSITY
- IHS2215
- Iii Mlch
- III nuistery of such superficialities was meant to
- Implement classifiers KMeans, Random Forest and Decision Tree, SVM,XGBoost and Naive Bayes for the given dataset of audio samples to findout top genre for an audio sample(which one fits best)
- In a cardiac issue what are the key steps necessary to include for prevention and health promotion.
- in any other way
- include a paragraph about which side of the case a forensic psychologists might support and why.
- indeed
- India
- INDIANA UNIVERSITY BLOOMINGTON, SCIENCE
- INF690
- INF690, Other
- Information Systems
- Information SystemsInformation Systems
- Information Technology
- INSTITUTE OF PUBLIC ADMINISTRATION SAUDI ARABIA, PROGRAMMING
- INT700, OTHER
- International Business
- International Relations
- International Studies
- Internet
- Introduction to Biology
- Is jury nullification sometimes justifiable? When?
- ISSC351
- It Research
- IT380
- IT550, Business & Finance , Southern New Hampshire University
- ITC3001
- ITP120
- ITS 631, PROGRAMMING
- ITS835, Other
- JEDDAH COLLEGE OF ADVERTISING, WRITING
- Journalism
- KNOWLEDGE IS POWER, OTHER
- Languages
- Law
- Law – Civil
- Law – CivilLaw – Civil
- Law – Criminal
- Law – CriminalLaw – Criminal
- LawLaw
- Leadership
- lecture slides
- Leisure Management
- Liberty University
- LIBERTY UNIVERSITY, WRITING
- lIlgll.Igt· (1II1In·d
- Linguistics
- literacy is always plural: literacies (there are many of them
- Literature
- Literature Review
- Literature review funnel on "cyber security"
- LiteratureLiterature
- MA105
- MAJAN COLLEGE, WRITING
- Management
- Manpower
- Marketing
- Math
- MATH 1030
- MATH144, MATHEMATICS
- Mathematics
- Mathematics – Algebra
- Mathematics – Calculus
- Mathematics – Geometry
- Mathematics – Numerical analysis
- Mathematics – Precalculus
- Mathematics – Probability
- Mathematics – Statistics
- Mathematics – StatisticsMathematics – Statistics
- Mathematics – Trigonometry
- MATHEMATICS, MGT3332
- Mathematics, National American University
- Mathematics, PSY325
- MATHEMATICS, PUBH8545
- Mathematics, QNT275
- MATHEMATICS, STAT 201
- MBA503
- McMaster University
- ME350B, SCIENCE
- MECH4430, SCIENCE
- Mechanics
- Media
- Medical
- Medical Essays
- MGMT2702
- MGMT410
- MGT173, SCIENCE
- MHR6451
- MIAMI UNIVERSITY, WRITING
- Military
- Military Science
- MKT501
- MKT690, OTHER
- MN576
- MN581
- MN610, SCIENCE
- MNGT3711
- Music
- MVC109
- N4685
- NATIONAL INSTITUTES OF HEALTH, SCIENCE
- NATIONAL UNIVERSITY, SCIENCE
- Needs to be at least 300 wordswithin the past five years.No plagiarism! What key aspects do you believe should guide ethical behavior related to health information, technology, and social media?
- no workable ""affirmative action"" for Discourses: you can't 19 Ill' let into the game after missing the apprenticeship and be expected to have a fnir shot at playing it. Social groups will not
- Northcentral University
- not writing)
- nothing can stand in her way once she has her mind set. I will say that she can sometimes be hard headed
- Nova Southeastern University
- NR447, SCIENCE
- NRS429VN
- NRS44V, OTHER
- NRS451VN
- NRSE4540
- NSG426
- NSG486
- NSG6102
- NSG6102, SCIENCE
- Numerical Analysis
- NUR231NUR2349, SCIENCE
- NUR647E
- NURS350
- NURS508
- NURS6640
- Nursing
- NURSING LEADERSHIP AND MANAGEMENT, SCIENCE
- NursingNursing
- Nutrition
- offering learners the flexibility to acquire new skills and knowledge from the comfort of their homes. However
- OHIO UNIVERSITY, SCIENCE
- Online Discussion Forums Grade and Reflection Assignment : Current Topic Artificial Intelligence HR Planning Career and Management Development Labour RelationsForum
- operation security
- Operations Management
- or do those companies have an ethical obligation to protect people? In this assignment
- ORG5800, OTHER
- Organisations
- OTHER
- Other, PAD631
- OTHER, PARK UNIVERSITY
- OTHER, PLA1223
- Other, POLI330N
- OTHER, PROFESSIONAL NURSING NU231 NUR2349
- Other, RTM404
- OTHER, SAINT LEO UNIVERSITY
- OTHER, SOC3210C1
- Other, SOCW6333
- OTHER, SOUTHERN NEW HAMPSHIRE UNIVERSITY
- Other, The University Of Southern Mississippi
- OTHER, TRIDENT UNIVERSITY INTERNATIONAL
- Other, UC
- OTHER, UNIVERSITY OF MARYLAND UNIVERSITY COLLEGE
- OTHER, UNIVERSITY OF SOUTH FLORIDA
- Other, Walden University
- paying attention to grammar and spelling. Actively participate in discussions
- Personal Development
- PhD Dissertation Research
- PHI 413V, SCIENCE
- Philosophy
- Photography
- PHY290
- PHYS204L206
- Physics
- Physics – Astronomy
- Physics – Electromagnetism
- Physics – Geophysics
- Physics – Mechanics
- Physics – Optics
- PhysicsPhysics
- Physiology
- PNGE332, SCIENCE
- Political Science
- Political SciencePolitical Science
- Politics
- PowerPoint slides
- privacy
- PROFESSIONAL NURSING NU231 NUR2349, SCIENCE
- PROFESSIONAL NURSING NU231NUR2349, SCIENCE
- Programming
- Programming , College of Applied Sciences
- PROGRAMMING, STRAYER UNIVERSITY
- PROGRAMMING, WILMINGTON UNIVERSITY
- Project Management
- proper grammar
- Protein
- provide a discussion on what could have been done better to minimize the risk of failure. If you have not yet been involved with a business process redesign
- PSYC8754, WRITING
- Psychology
- PsychologyPsychology
- PUB373, SCIENCE
- Purdue University
- Rasmussen College
- Read a poam and write a paragraph to prove "The table turned".
- Reading
- ReadingReading
- readings
- Reference this
- REL1030
- Religion
- RES861, Science RES861
- Research Methodology
- Research methods
- Research Proposal
- Research questions
- Retail
- Rutgers university
- SAFE4150
- safety statutes
- Santa Clara University
- SCI 220, SCIENCE
- SCI115, SCIENCE
- Science
- Science, Strayer University
- SCIENCE, THOMAS JEFFERSON UNIVERSITY
- SCIENCE, WEST COAST UNIVERSITY
- SCIENCE, WEST TEXAS A & M UNIVERSITY
- Sciences
- SCM371, Writing
- Search in scholarly sources the similarities and difference between PhD and DNP. Post three similarities and three differences found on your research. Do not forget to include your reference.
- Security
- self-actualization
- several things can happen
- Should the government operate public transportation systems?250 words
- so that it is not biased?
- so too
- SOC 450
- Social Policy
- Social Science
- Social Science – Philosophy
- Social Science – PhilosophySocial Science – Philosophy
- Social Science – Sociology
- Social Science – SociologySocial Science – Sociology
- Social Sciences
- Social ScienceSocial Science
- Social Work
- Society
- Sociology
- someone cannot engage in a Discourse in a less than fully fluent manner. You are either in it or you're not. Discourses are connected with displays of
- SP19, WRITING
- SPC2608
- SPD310
- Sports
- Statistics
- succeeding in online courses requires a different approach compared to traditional classroom settings. To help you make the most of your online learning experience
- such as notifications from social media or email. Organize your study materials and have a reliable internet connection to ensure seamless access to course materials.
- Technology
- that personal ethics and organizations ethics are two different and unrelated concepts. Others
- the attribute is useful
- The directions are attached. However you must read the PDF file first in order to answer the questions.
- the role of work and money
- Theatre
- then reply to a minimum of 2 of your classmates' original posts.
- Theology
- Threat of artificial intelligence 800 words.
- to be true of second language acquisition or socially situ ated cognition (Beebe
- to better promote the value and dignity of individuals or groups and to serve others in ways that promote human flourishing.
- to usc a Discourse. The most you can do is III It'! them practice being a linguist with you.
- total fat consumption
- Tourism
- Translation
- Transportation
- U110
- Uncategorized
- University of Central Missouri
- University of South Florida
- UNIVERSITY OF SOUTH FLORIDA, WRITING
- Video Games
- Watch this meditation https://www.youtube.com/watch?v=doQGx4hdF3M&feature=youtu.be and write a one page reflection
- WCWP10B
- we can always ask about how much ten- 12 """""" or conflict is present between any two of a person's Discourses (Rosaldo
- What approaches to the study of poverty does economic sociology offer? More specifically, what might sociologists studying poverty focus on besides poor households, neighborhoods, and individuals?
- What is the philosophical matrices for Behaviorism, Constructivism, and Reconstructivism
- What key aspects do you believe should guide ethical behavior related to health information, technology, and social media?
- what place did government have to improve markets? What does the concept of ""crowding out"" mean in all of this?
- What should be done to maintain optimum stock levels and why is it important to keep accurate and up-to-date records of stock in medical practice?
- whether good or bad. The intent of the short research projects is to dig a little deeper into some of the topics
- which triggers the vulnerability. As soon as the user downloads this shortcut file on Windows 10; windows explorer will
- Would somebody read these quotes and answer those three questions at the bottom for me?Disregard number two I will look for myself in the text.
- Write short essay based on evidence about the 2 cons of Sex Education 250-300 words 2 reference minimum no plagiarism
- WRITING
- writing assignment, you will analyze asymmetric and symmetric encryption. Evaluate the differences between the two of them and which one that you would determine is the most secure.
- Writing, Personal Code of Technology Ethics
- you believe you can provide the CIO with the information he needs.
- you will learn how to search for scholarly
- you will need to read the TCP standard. TCP was first defined in RFC 793. A link to this document is provided. https://tools.ietf.org/html/rfc793
- Young People
Recent Comments